Multi-group image supervised classification method based on empirical mode decomposition
An empirical modal decomposition and supervised classification technology, applied in the field of image processing, can solve the problems of low classification accuracy and insufficient feature utilization, and achieve the effect of good consistency and improved classification accuracy.
- Summary
- Abstract
- Description
- Claims
- Application Information
AI Technical Summary
Problems solved by technology
Method used
Image
Examples
specific Embodiment approach 1
[0023] Specific implementation mode one: the following combination Figure 1 to Figure 10 Describe this implementation mode, this implementation mode comprises the following steps:
[0024] Step 1: Carry out empirical mode decomposition to the training eigenvector formed by each pixel characteristic curve of the training vector in the multi-group image, and obtain the extended training eigenvector of each pixel of the training vector;
[0025] Carry out empirical mode decomposition to the test feature vector formed by each pixel characteristic curve of the test vector in the multi-group image, and obtain the extended test feature vector of each pixel of the test vector;
[0026] The method to obtain the extended training feature vector or the extended test feature vector is:
[0027] Set the input vector signal as x(t), where 1≤t≤N, N is the total number of bands of the multi-group image,
[0028] set r n (t) is the residual trend function of the nth eigenmode function deco...
specific Embodiment approach
[0073] Hyperspectral image is one of the typical multi-group images. The 92AV3C hyperspectral image comes from the remote sensing observation of an agricultural area in northwestern Indiana, USA by the AVIRIS (Airborne Visible / Infrared Imaging Spectrometer) sensor. The data set contains 220 bands (the other 4 bands are all 0 and discarded), from 0.40 μm to 2.45 μm approximately every 10nm, and a reference map of each pixel’s category calibrated through field investigation is attached. This reference image can be used to assist in constructing a training set and calculating the classification accuracy of the classification method with respect to the test set. In this embodiment, the 7 categories with the most pixels in the 92AV3C hyperspectral image are arranged, which provides a sufficient data basis for the cross-validation experiment. The experiment uses up to 5-fold cross validation (5-fold cross validation) experiment to calculate the classification accuracy, which makes ...
specific Embodiment approach 2
[0093] Embodiment 2. The difference between this embodiment and Embodiment 1 is that T=0.25, and the others are the same as Embodiment 1.
PUM
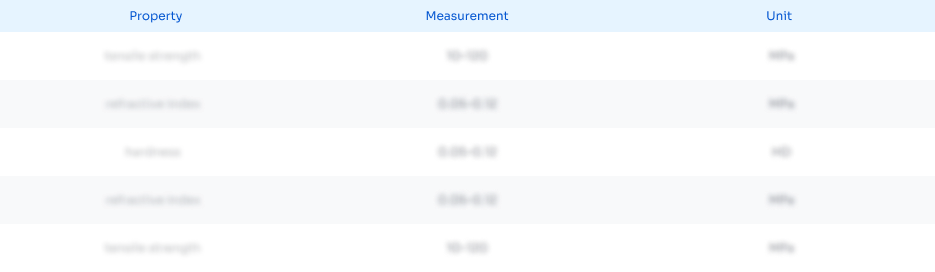
Abstract
Description
Claims
Application Information
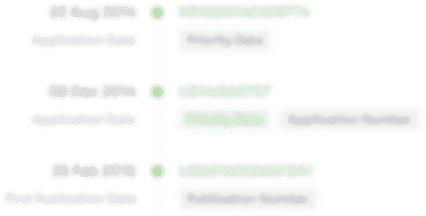
- R&D Engineer
- R&D Manager
- IP Professional
- Industry Leading Data Capabilities
- Powerful AI technology
- Patent DNA Extraction
Browse by: Latest US Patents, China's latest patents, Technical Efficacy Thesaurus, Application Domain, Technology Topic.
© 2024 PatSnap. All rights reserved.Legal|Privacy policy|Modern Slavery Act Transparency Statement|Sitemap