Graph-based semi-supervised high-spectral remote sensing image classification method
A technology of hyperspectral remote sensing and classification method, which is applied in the field of graph-based semi-supervised hyperspectral remote sensing image classification, which can solve the problems of difficult classification effects and difficult to obtain classification results, and achieve the effect of small restrictions
Active Publication Date: 2011-06-15
XIDIAN UNIV
View PDF5 Cites 84 Cited by
- Summary
- Abstract
- Description
- Claims
- Application Information
AI Technical Summary
Problems solved by technology
For the classification of hyperspectral remote sensing images, due to the small number of marked sample points, it is difficult to obtain better classification results using traditional supervised learning algorithms, and it is difficult to obtain better classification results without using label information in unsupervised methods classification result
Method used
the structure of the environmentally friendly knitted fabric provided by the present invention; figure 2 Flow chart of the yarn wrapping machine for environmentally friendly knitted fabrics and storage devices; image 3 Is the parameter map of the yarn covering machine
View moreImage
Smart Image Click on the blue labels to locate them in the text.
Smart ImageViewing Examples
Examples
Experimental program
Comparison scheme
Effect test
Embodiment 1
Embodiment 2
Embodiment 3
the structure of the environmentally friendly knitted fabric provided by the present invention; figure 2 Flow chart of the yarn wrapping machine for environmentally friendly knitted fabrics and storage devices; image 3 Is the parameter map of the yarn covering machine
Login to View More PUM
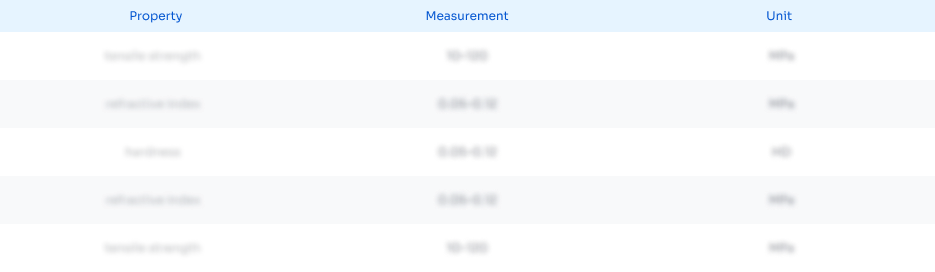
Abstract
The invention relates to a graph-based semi-supervised high-spectral remote sensing image classification method. The method comprises the following steps: extracting the features of an input image; randomly sampling M points from an unlabeled sample, constructing a set S with L marked points, constructing a set R with the rest of the points; calculating K adjacent points of the points in the sets S and R in the set S by use of a class probability distance; constructing two sparse matrixes WSS and WSR by a linear representation method; using label propagation to obtain a label function F<*><S>, and calculating the label prediction function F<*><R> of the sample points in the set R to determine the labels of all the pixel points of the input image. According to the method, the adjacent points of the sample points can be calculated by use of the class probability distance, and the accurate classification of high-spectral images can be achieved by utilizing semi-supervised conduction, thus the calculation complexity is greatly reduced; in addition, the problem that the graph-based semi-supervised learning algorithm can not be used for large-scale data processing is solved, and the calculation efficiency can be improved by at least 20-50 times within the per unit time when the method provided by the invention is used, and the visual effects of the classified result graphs are good.
Description
Graph-based semi-supervised hyperspectral remote sensing image classification method technical field The invention belongs to the technical field of image processing and relates to the classification of hyperspectral remote sensing images, which can be used for preprocessing hyperspectral remote sensing images, in particular to a graph-based semi-supervised hyperspectral remote sensing image classification method. Background technique Hyperspectral remote sensing images have high spectral resolution and provide rich information about the types of ground objects. The classification of remote sensing images is one of the key technologies for the analysis and application of remote sensing images. How to deal with the massive data and high-dimensional characteristics of hyperspectral images and combine various features of hyperspectral images to study fast and efficient target recognition and classification algorithms It is a hotspot in hyperspectral image processing research ...
Claims
the structure of the environmentally friendly knitted fabric provided by the present invention; figure 2 Flow chart of the yarn wrapping machine for environmentally friendly knitted fabrics and storage devices; image 3 Is the parameter map of the yarn covering machine
Login to View More Application Information
Patent Timeline
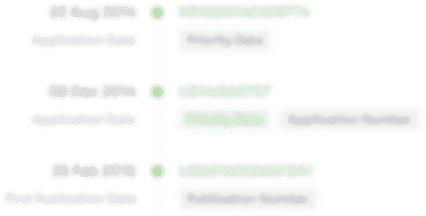
IPC IPC(8): G06K9/62
Inventor 张向荣焦李成魏征丽侯彪李阳阳杨淑媛刘若辰马文萍
Owner XIDIAN UNIV
Features
- Generate Ideas
- Intellectual Property
- Life Sciences
- Materials
- Tech Scout
Why Patsnap Eureka
- Unparalleled Data Quality
- Higher Quality Content
- 60% Fewer Hallucinations
Social media
Patsnap Eureka Blog
Learn More Browse by: Latest US Patents, China's latest patents, Technical Efficacy Thesaurus, Application Domain, Technology Topic, Popular Technical Reports.
© 2025 PatSnap. All rights reserved.Legal|Privacy policy|Modern Slavery Act Transparency Statement|Sitemap|About US| Contact US: help@patsnap.com