Cross-domain video semantic concept detection method based on active learning
An active learning and detection method technology, applied in the field of cross-domain video semantic concept detection, can solve the problem of different feature space distribution, and achieve the effect of reducing sample complexity, time complexity, and high classification accuracy
- Summary
- Abstract
- Description
- Claims
- Application Information
AI Technical Summary
Problems solved by technology
Method used
Image
Examples
Embodiment Construction
[0032] The present invention proposes a cross-domain video semantic concept detection method based on active learning, using Gaussian random field as a benchmark classifier, and using marked original domain data and unmarked target domain data as training data, according to The most uncertain principle in the active learning query strategy selects samples for labeling, adds their new labels to the Gaussian random field, updates the model, and then re-selects the new most uncertain samples for labeling, which specifically includes the following steps:
[0033] exist figure 1 In the flow chart of the cross-domain active learning algorithm of the present invention, the present invention mainly includes four steps of using a Gaussian random field as a benchmark classifier, selecting samples according to the principle of most uncertainty for labeling, and updating the benchmark classifier.
[0034] Step 1: Use a Gaussian random field as a baseline classifier
[0035] Treat each sa...
PUM
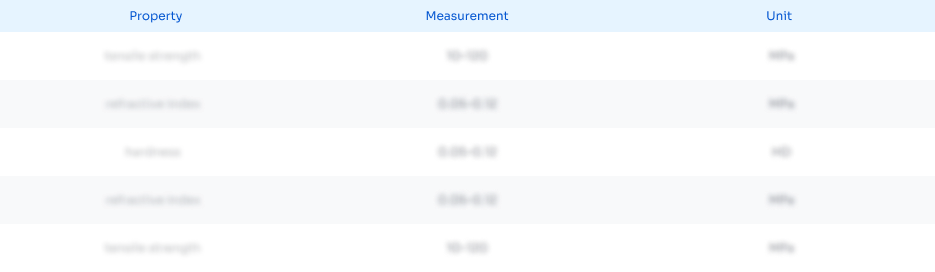
Abstract
Description
Claims
Application Information
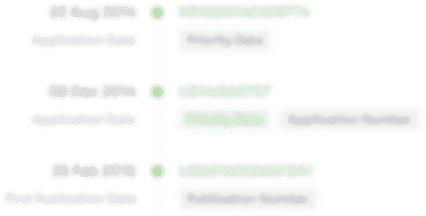
- Generate Ideas
- Intellectual Property
- Life Sciences
- Materials
- Tech Scout
- Unparalleled Data Quality
- Higher Quality Content
- 60% Fewer Hallucinations
Browse by: Latest US Patents, China's latest patents, Technical Efficacy Thesaurus, Application Domain, Technology Topic, Popular Technical Reports.
© 2025 PatSnap. All rights reserved.Legal|Privacy policy|Modern Slavery Act Transparency Statement|Sitemap|About US| Contact US: help@patsnap.com