Relevance vector machine-based multi-class data classifying method
A correlation vector machine and data classification technology, applied in the field of data processing, can solve the problems of less total time, overlapping classification, unsuitability, etc., and achieve the effect of variable test time, clear classification basis, and simple classification interface
- Summary
- Abstract
- Description
- Claims
- Application Information
AI Technical Summary
Problems solved by technology
Method used
Image
Examples
Embodiment Construction
[0045] refer to figure 1 , the identification and refusal steps of the multi-category data classification method of the present invention are as follows:
[0046] Step 1. Divide the multi-class data set into cross-validation data set V, training data set R and test data set T, and perform normalization preprocessing on them so that different features of the data are on the same scale.
[0047] The multi-class data set is divided as follows: a part of the samples or all samples in the multi-class data set are used as the cross-validation data set V, and then the remaining samples or all samples in the multi-class data set are divided according to the number of samples in the training data set R and the test data The ratio of the number of samples in set T is 2:3 for division.
[0048] The features of different dimensions of the data are responses to different aspects of the target, and their representation scales may not be on the same order of magnitude, and it is im...
PUM
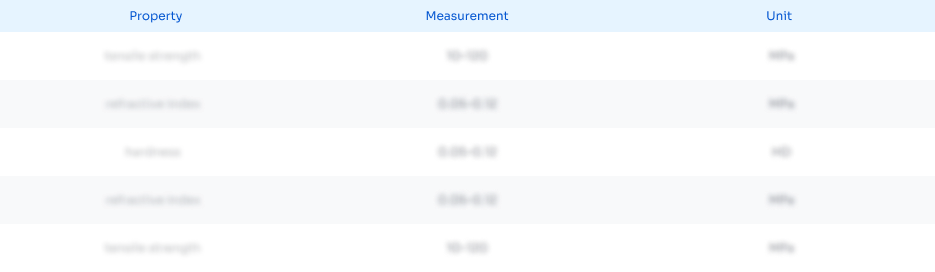
Abstract
Description
Claims
Application Information
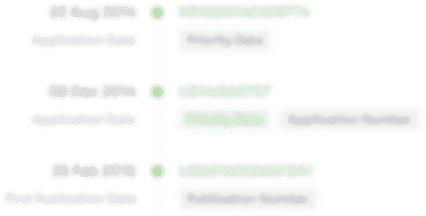
- R&D Engineer
- R&D Manager
- IP Professional
- Industry Leading Data Capabilities
- Powerful AI technology
- Patent DNA Extraction
Browse by: Latest US Patents, China's latest patents, Technical Efficacy Thesaurus, Application Domain, Technology Topic, Popular Technical Reports.
© 2024 PatSnap. All rights reserved.Legal|Privacy policy|Modern Slavery Act Transparency Statement|Sitemap|About US| Contact US: help@patsnap.com