A Robust Speech Feature Extraction Method Based on Sparse Decomposition and Reconstruction
A sparse decomposition, speech feature technology, applied in speech analysis, speech recognition, instruments, etc., can solve the problem of ignoring the probability of mutual conversion of atomic prior probabilities
- Summary
- Abstract
- Description
- Claims
- Application Information
AI Technical Summary
Problems solved by technology
Method used
Image
Examples
specific Embodiment approach 1
[0027] Specific implementation mode one: combine figure 1 Describe this implementation mode, this implementation mode comprises concrete steps as follows:
[0028] Step 1, preprocessing, divide the read-in speech into frames and add windows, so that the speech is converted from a time sequence to a frame sequence;
[0029] Step 2. Perform discrete Fourier transform and calculate the power spectrum: X a ( k ) = | | Σ n = 0 N - 1 x ( n ) e - j 2 kπ ...
specific Embodiment approach 2
[0039] Specific implementation mode two: the specific process of step one in the implementation mode one is:
[0040] The input of the present invention is a discrete-time signal of speech, and the speech must be preprocessed first, including framing and windowing. The purpose of framing is to divide the time signal into overlapping speech segments, i.e. frames; next, add a window to each frame of speech; the window functions widely used at present have Hamming window and Hanning window, and the present invention adopts Hamming window window:
[0041]
[0042] Among them, n is the time sequence number, and L is the window length. Other steps are the same as those in Embodiment 1.
specific Embodiment approach 3
[0043] Specific implementation mode three: the specific process of step three in the first implementation mode is: select a representative frame from the training speech frame as an atom under the condition that the error of the reconstructed training sample is the smallest; for the noise atom, consider dynamic update, To track the influence of time-varying noise, Algorithm I is proposed:
[0044] Algorithm I
[0045]
[0046] where Φ is the atom dictionary, d(f t , Φ)=min{d i |d i =||f t -φ i || 2}; where φ i is the i-th atom in the current Φ, ||·|| 2 is the 2-norm operator; the algorithm first empty the atom dictionary, define d(f t, φ)=0, φ represents an empty set; then starting from the first frame of speech, add atoms one by one according to the minimum distance criterion, and discard the speech frames that are very similar to the atoms in the atom dictionary in the remaining speech frames, otherwise, add Atom dictionary; this algorithm can ensure that the sig...
PUM
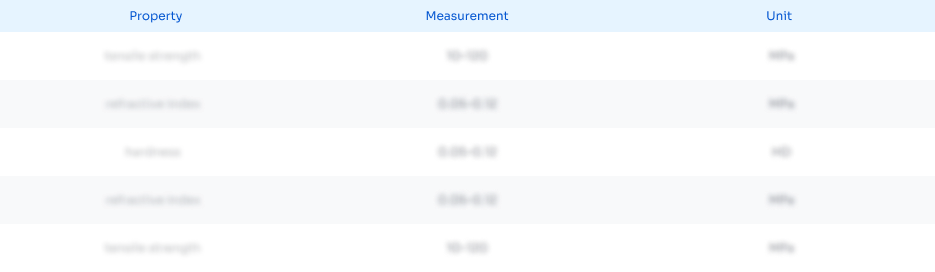
Abstract
Description
Claims
Application Information
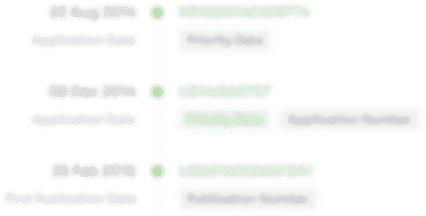
- R&D Engineer
- R&D Manager
- IP Professional
- Industry Leading Data Capabilities
- Powerful AI technology
- Patent DNA Extraction
Browse by: Latest US Patents, China's latest patents, Technical Efficacy Thesaurus, Application Domain, Technology Topic, Popular Technical Reports.
© 2024 PatSnap. All rights reserved.Legal|Privacy policy|Modern Slavery Act Transparency Statement|Sitemap|About US| Contact US: help@patsnap.com