Total variation and euler elastic rod-based supervised mode identification method
A technology of pattern recognition and total variation, applied in the field of pattern recognition, which can solve the problems of inconsistent actual distribution, difficulty in effectively estimating the probability density function of data, and penalizing the gap width of the interface.
- Summary
- Abstract
- Description
- Claims
- Application Information
AI Technical Summary
Problems solved by technology
Method used
Image
Examples
Embodiment 1
[0030] Example 1: USPS handwritten digit recognition
[0031] The USPS (U.S.Postal Service) dataset uses handwritten digital pictures scanned on US postal envelopes. Each picture is a 16*16 grayscale picture, containing a number, such as figure 2 (a) shows part of its data display, from 0 to 9, a total of 10 categories. In this embodiment, 1000 samples were randomly selected for experiments. Since the original data had a high dimension, principal component analysis (PCA) was used to reduce it to 30 dimensions and normalize it to the [0, 1] interval.
[0032] Step 1: Construct the energy functional under the regularization framework
[0033] (a) Total variation energy functional
[0034] Mathematically, the Total Variation of the function f(x) has the form in the one-dimensional case and when the function is continuous: Represents the total variation of f(x), a and b are the endpoints of the interval, and f'(x) is the derivative of the function. It can be seen that tota...
Embodiment 2
[0089] Embodiment 2: Applied to some other common classification data
[0090] This embodiment is based on the data sets of 8 classifications in the libsvm website and the UCI machine learning library, including two types and multiple types of data. For all data sets are normalized to the interval [0, 1], the operation steps are the same as those in the first embodiment. For all methods, five-fold cross-validation is still used to select the optimal parameters. The comparison of the optimal accuracy of each method is shown in Table 3:
[0091] Table 3 Comparison of classification accuracy of each method (%)
[0092]
[0093] As can be seen from Table 3, the IagLE solution of the TV and EE pattern recognition methods proposed by the present invention is generally better than that of GD, and the effect of EE is slightly better than that of TV, and they are all more accurate than BPNN on all data sets to be tall. The EE method surpassed the SVM on six data sets under the Ia...
PUM
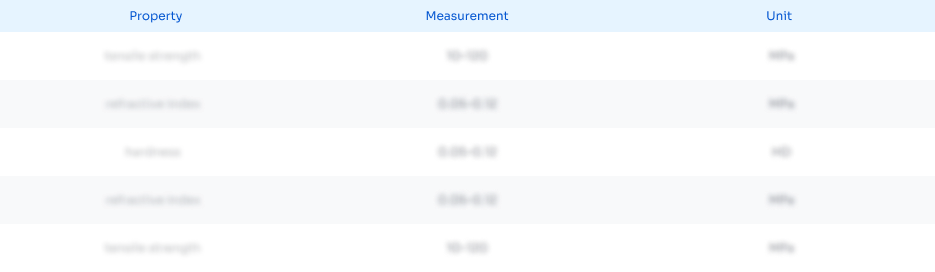
Abstract
Description
Claims
Application Information
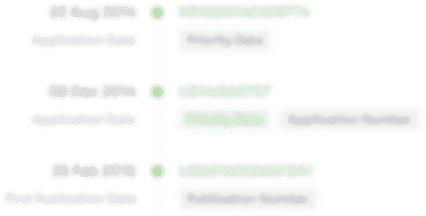
- R&D Engineer
- R&D Manager
- IP Professional
- Industry Leading Data Capabilities
- Powerful AI technology
- Patent DNA Extraction
Browse by: Latest US Patents, China's latest patents, Technical Efficacy Thesaurus, Application Domain, Technology Topic, Popular Technical Reports.
© 2024 PatSnap. All rights reserved.Legal|Privacy policy|Modern Slavery Act Transparency Statement|Sitemap|About US| Contact US: help@patsnap.com