Self-adaptive parameter soft measuring method on basis of semi-supervised local linear regression
A self-adaptive parameter, local linear technology, applied in the field of soft measurement instruments, can solve the problems of unlabeled samples and the model cannot be effectively updated, and achieve the effect of improving prediction accuracy and wide application prospects.
- Summary
- Abstract
- Description
- Claims
- Application Information
AI Technical Summary
Problems solved by technology
Method used
Image
Examples
Embodiment
[0027] Such as figure 1 As shown, this embodiment includes the following steps: First, initialize the labeled data set X L , all data sets X, prediction set Initialize time window width and semi-supervised coefficientγ 0 and Gaussian kernel width h 0 ; Then read the input data, judge whether it is a labeled sample, update the data set, and select an appropriate Gaussian kernel width h according to the adaptive parameter selection method suitable for semi-supervised local linear regression; finally use h to perform semi-supervised local linear regression soft The measurement model is built and the resulting prediction is updated, and the prediction set is updated, and the cycle continues until the algorithm terminates.
[0028] Input: labeled sample set X L , all data sets X.
[0029] ①Initialize coefficient matrix B, prediction set Select the rolling window size and create a labeled data set X L , all data sets X, set appropriate h 0 and gamma 0 .
[0030] ② Read d...
PUM
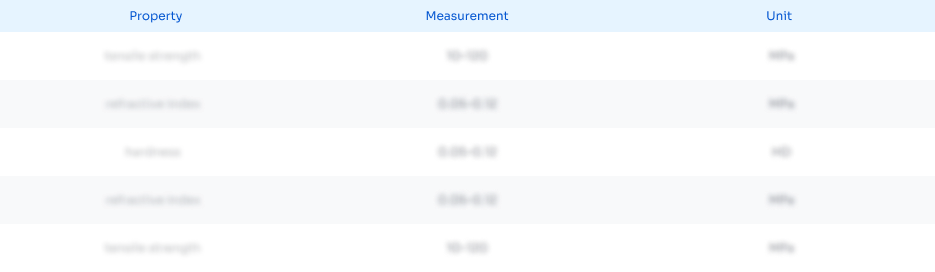
Abstract
Description
Claims
Application Information
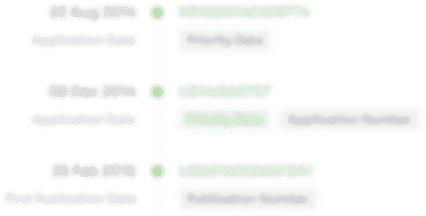
- R&D Engineer
- R&D Manager
- IP Professional
- Industry Leading Data Capabilities
- Powerful AI technology
- Patent DNA Extraction
Browse by: Latest US Patents, China's latest patents, Technical Efficacy Thesaurus, Application Domain, Technology Topic, Popular Technical Reports.
© 2024 PatSnap. All rights reserved.Legal|Privacy policy|Modern Slavery Act Transparency Statement|Sitemap|About US| Contact US: help@patsnap.com