Face image dimension reducing method based on local correlation preserving
A technology of local association preservation and face image, applied in the field of face image dimensionality reduction, it can solve the problems of not being able to directly obtain the transformation features of unlabeled face images, not giving a nonlinear transformation matrix, and complicated calculation, so as to reduce the computational complexity. Complexity, the effect that is beneficial to image recognition
- Summary
- Abstract
- Description
- Claims
- Application Information
AI Technical Summary
Problems solved by technology
Method used
Image
Examples
Embodiment Construction
[0024] The present invention will be further described below in conjunction with the accompanying drawings and embodiments.
[0025] like figure 1 Shown is the flow chart of the dimensionality reduction method of the present invention, a face image dimensionality reduction method based on local association preservation,
[0026] The specific steps are:
[0027] Step 1: Express m face images with a size of s×t pixels as a row vector x of s×t dimension 1 ,x 2 ,...,x i ,...,x m , where m is the number of face images, s is the number of image row pixels, t is the number of column image column pixels, x i Represents the s×t-dimensional row vector corresponding to the i-th face image, these m face images contain p people, each person images;
[0028] Step 2: For any row vector x i (i∈{1,2,…,m}), calculate d ij =‖x i -x j ‖(j∈{1,2,…,m} and j≠i), select k from them (k=9) such that d ij The smallest row vector, the composition set is recorded as Ne(x i ). Where ‖·‖ repre...
PUM
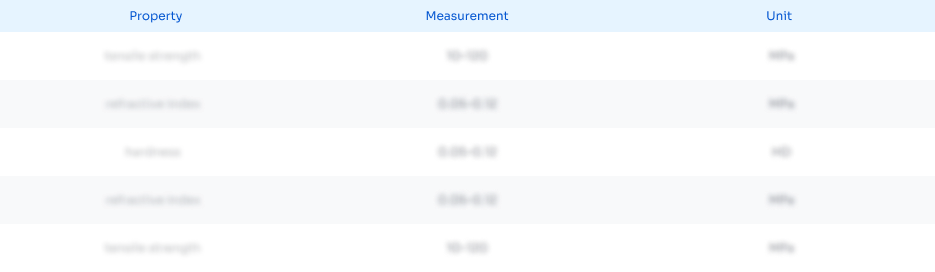
Abstract
Description
Claims
Application Information
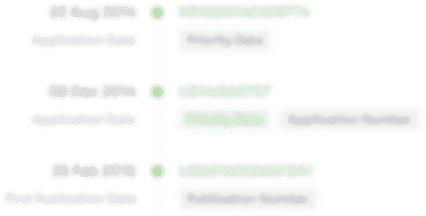
- R&D
- Intellectual Property
- Life Sciences
- Materials
- Tech Scout
- Unparalleled Data Quality
- Higher Quality Content
- 60% Fewer Hallucinations
Browse by: Latest US Patents, China's latest patents, Technical Efficacy Thesaurus, Application Domain, Technology Topic, Popular Technical Reports.
© 2025 PatSnap. All rights reserved.Legal|Privacy policy|Modern Slavery Act Transparency Statement|Sitemap|About US| Contact US: help@patsnap.com