Probabilistic Refinement for Model-Based Segmentation
A probabilistic and model-based technology, applied in the field of image segmentation, which can solve problems such as multiple potential solutions, unfavorable structures or organ boundaries, and achieve reliable segmentation results.
- Summary
- Abstract
- Description
- Claims
- Application Information
AI Technical Summary
Problems solved by technology
Method used
Image
Examples
Embodiment Construction
[0021] refer to figure 1 , a diagnostic imaging scanner 10 such as a CT scanner, MRI scanner, PET scanner, nuclear scanner, ultrasound scanner, etc. generates image data which is reconstructed by a reconstruction processor 12 to generate a current 3D diagnostic image, The current 3D diagnostic image is stored in memory, storage segment or buffer 14 .
[0022] continue to refer figure 1 , and further reference figure 2 , the memory or storage segment 20 stores a library of 3D probability maps 22 . The probability map defines the volume of the study region 24, which is known to be the portion of the region or volume under study, ie the brainstem in the present example. Background region 26 defines objects or tissue known as background, ie non-brainstem. That is, voxels in the brainstem region 24 have a 100% probability of delineating the brainstem and a 0% probability of delineating the background. In contrast, voxels in the background region 26 have a 100% probability of ...
PUM
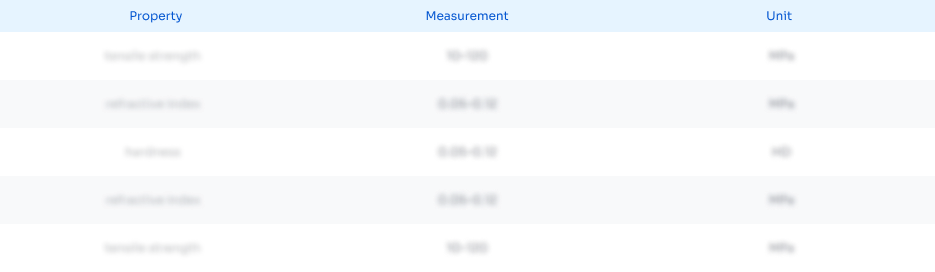
Abstract
Description
Claims
Application Information
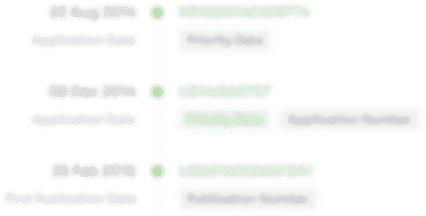
- R&D Engineer
- R&D Manager
- IP Professional
- Industry Leading Data Capabilities
- Powerful AI technology
- Patent DNA Extraction
Browse by: Latest US Patents, China's latest patents, Technical Efficacy Thesaurus, Application Domain, Technology Topic, Popular Technical Reports.
© 2024 PatSnap. All rights reserved.Legal|Privacy policy|Modern Slavery Act Transparency Statement|Sitemap|About US| Contact US: help@patsnap.com