Aurora image classification method based on biological stimulation characteristic and manifold learning
A classification method and manifold learning technology, applied in the field of image processing, can solve the problems of ineffective classification of aurora images and time-consuming, and achieve the effect of reducing computational complexity, strong robustness, and preserving differences
- Summary
- Abstract
- Description
- Claims
- Application Information
AI Technical Summary
Problems solved by technology
Method used
Image
Examples
Embodiment Construction
[0033] Attached below figure 1 The steps of the present invention are further described in detail.
[0034] Step 1, input the aurora image, and use the mask processing method to preprocess the input aurora image for edge denoising.
[0035] 1.1) Construct a binary image P with the same size as the aurora image, take the center of the binary image as the center, and make a circle with a radius of 220. The pixels inside the circle take a value of 1, and the pixels outside the circle take a value of 0 ;
[0036] 1.2) According to the constructed binary image P, mask the input aurora image, and use the following formula to obtain the preprocessed aurora image:
[0037] I=O*P,
[0038] Among them, I is the preprocessed aurora image, O is the original input aurora image, P is the circular mask image, and * is the multiplication operation of corresponding pixels between images.
[0039] Step 2, use the HMAX model to extract the C1 features of the aurora image.
[0040] 2.1) Cons...
PUM
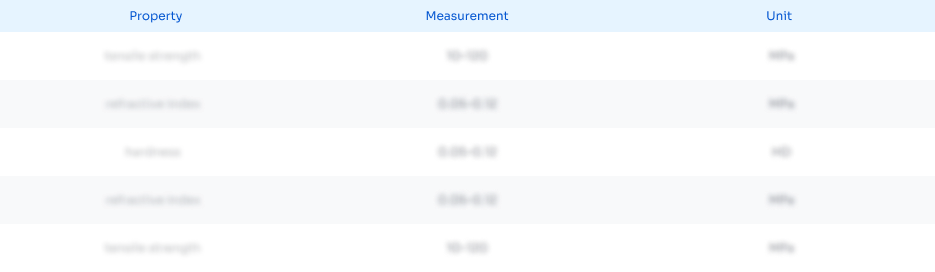
Abstract
Description
Claims
Application Information
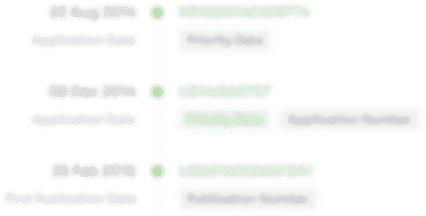
- R&D Engineer
- R&D Manager
- IP Professional
- Industry Leading Data Capabilities
- Powerful AI technology
- Patent DNA Extraction
Browse by: Latest US Patents, China's latest patents, Technical Efficacy Thesaurus, Application Domain, Technology Topic, Popular Technical Reports.
© 2024 PatSnap. All rights reserved.Legal|Privacy policy|Modern Slavery Act Transparency Statement|Sitemap|About US| Contact US: help@patsnap.com