FNN learning algorithm
A technology of fuzzy neural network and learning algorithm, applied in the design field of fuzzy neural network learning algorithm, can solve the problems such as the learning rate and step size cannot be changed, the data learning is insufficient, and the data cannot be recognized.
- Summary
- Abstract
- Description
- Claims
- Application Information
AI Technical Summary
Problems solved by technology
Method used
Image
Examples
Embodiment Construction
[0043] The present invention will be further elaborated below in conjunction with the accompanying drawings and specific embodiments.
[0044] Such as figure 1 Shown is a flow chart of a fuzzy neural network learning algorithm according to the embodiment of the present invention, which specifically includes the establishment of fuzzy neural network, the training of fuzzy neural network parameters, and the prediction and identification of the trained fuzzy neural network. As a result, the category to which the object belongs is judged. The invention increases the identification of data with higher fuzziness by learning and training the parameters of the fuzzy neural network, wherein the learning rate changes continuously with the learning process. Such as figure 2 Shown is the specific learning flowchart of a kind of fuzzy neural network learning algorithm of the embodiment of the present invention, below its specific steps are described in detail, a kind of fuzzy neural net...
PUM
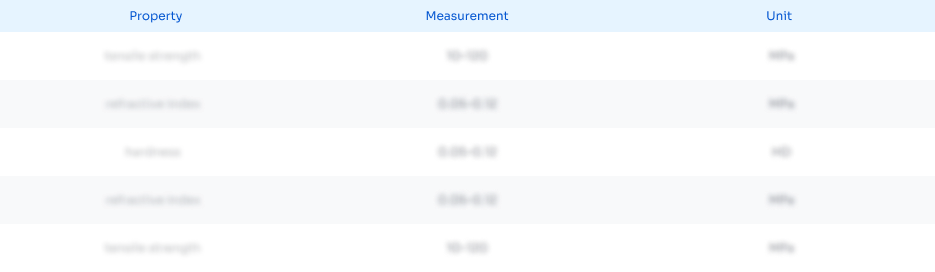
Abstract
Description
Claims
Application Information
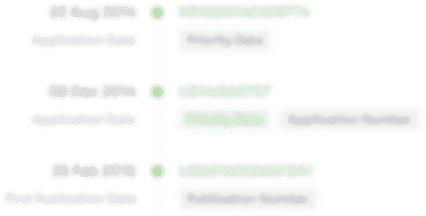
- R&D Engineer
- R&D Manager
- IP Professional
- Industry Leading Data Capabilities
- Powerful AI technology
- Patent DNA Extraction
Browse by: Latest US Patents, China's latest patents, Technical Efficacy Thesaurus, Application Domain, Technology Topic, Popular Technical Reports.
© 2024 PatSnap. All rights reserved.Legal|Privacy policy|Modern Slavery Act Transparency Statement|Sitemap|About US| Contact US: help@patsnap.com