Data flow concept drift detection method and system
A technology of concept drift and detection method, applied in the field of computer, which can solve the problems of incomplete detection, inability to comprehensively analyze, and the need for manual labor.
- Summary
- Abstract
- Description
- Claims
- Application Information
AI Technical Summary
Problems solved by technology
Method used
Image
Examples
Embodiment Construction
[0059] figure 1 It is a flow chart of the steps of a data flow concept drift detection method of the present invention, and a data flow concept drift detection method of the present invention includes the following steps:
[0060] A. According to the cluster set, the old data set and the data set to be detected, calculate the sum of the squares of the distances of the cluster tolerance point set of the old data set and the sum of the squares of the distances of the cluster tolerance point set of the data set to be detected;
[0061] B. Calculate the cluster evolution value of each cluster in the cluster according to the decay function and the data set to be detected;
[0062] C. Obtain the cluster intolerable point set corresponding to the data set to be detected through analysis and divide its data points to form a new cluster, and then calculate the new cluster acceptance value of each cluster in the new cluster;
[0063] D. Calculate the concept drift level value based on ...
PUM
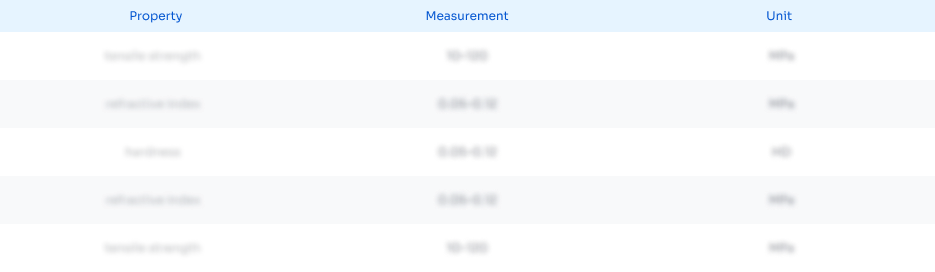
Abstract
Description
Claims
Application Information
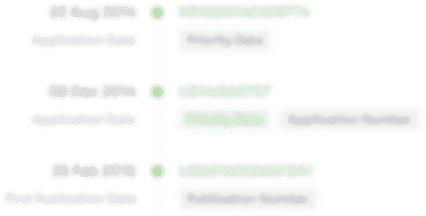
- R&D Engineer
- R&D Manager
- IP Professional
- Industry Leading Data Capabilities
- Powerful AI technology
- Patent DNA Extraction
Browse by: Latest US Patents, China's latest patents, Technical Efficacy Thesaurus, Application Domain, Technology Topic, Popular Technical Reports.
© 2024 PatSnap. All rights reserved.Legal|Privacy policy|Modern Slavery Act Transparency Statement|Sitemap|About US| Contact US: help@patsnap.com