Online sub-pixel optical component damage detection method based on radiation calibration
A technology of optical components and detection methods, which is applied in the direction of optical testing flaws/defects, etc., which can solve the problems of high-precision damage size, inability to obtain online detection images, etc.
- Summary
- Abstract
- Description
- Claims
- Application Information
AI Technical Summary
Problems solved by technology
Method used
Image
Examples
specific Embodiment approach 1
[0038] Specific Embodiment 1: The online detection method for sub-pixel optical element damage based on radiation calibration in this embodiment includes the following contents:
[0039]1. After obtaining the total gray level of all damaged areas of the optical element through the traditional online detection method, randomly select N damaged areas, obtain the accurate size of the damaged area in the offline detection system, and establish a sample set
[0040] D={(x 1 ,y 2 ),...., (x k ,y k ),...., (x N ,y N )}, x k ,y k ∈R; where x k is the total gray level of each damage area in the online image, y k High-precision size obtained under high-precision off-line system for the damage area;
[0041] 2. Estimate the probability of non-outlier points in the sample set ε, through the formula Calculate the number of groups M that need to be randomly sampled;
[0042] 3. Randomly select M groups of small samples from the sample set D {W 1 (x 1,1 ,y 1,1 ,...,x 1,I ,y ...
specific Embodiment approach 2
[0076] Specific embodiment 2: The difference between this embodiment and specific embodiment 1 is that the non-outlier probability ε in the step 2 is defined as:
[0077]
[0078] Generally, the exact value of ε of the sample cannot be obtained, but its approximate value can be obtained by analyzing the original data. Unlike the general RANSAC method, the minimum number of samples I used to establish the LSSVM model is only related to the quality of the sample, and the appropriate I can be obtained through experiments. . Other steps and parameters are the same as those in Embodiment 1.
specific Embodiment approach 3
[0079] Specific embodiment three: the difference between this embodiment and specific embodiment one or two is that a series of LSSVM regression models established in the step four are expressed as constrained optimization problems:
[0080] min w , b , e J ( ω , e ) = 1 2 | | ω | | 2 + γ 2 Σ k = 1 N e k 2
[0081]
[0082] in is a nonlinear mapping function, transforming the linear inseparable problem into a linear problem in high-dimensiona...
PUM
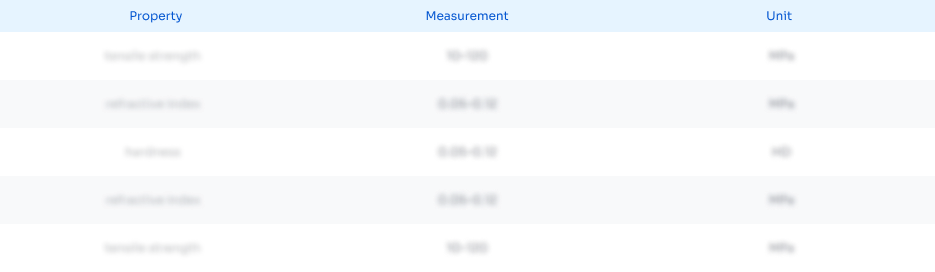
Abstract
Description
Claims
Application Information
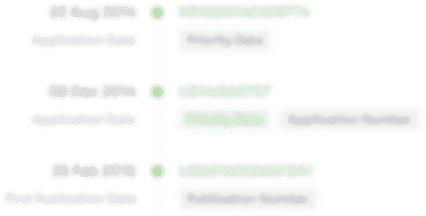
- R&D Engineer
- R&D Manager
- IP Professional
- Industry Leading Data Capabilities
- Powerful AI technology
- Patent DNA Extraction
Browse by: Latest US Patents, China's latest patents, Technical Efficacy Thesaurus, Application Domain, Technology Topic, Popular Technical Reports.
© 2024 PatSnap. All rights reserved.Legal|Privacy policy|Modern Slavery Act Transparency Statement|Sitemap|About US| Contact US: help@patsnap.com