Integrated framework method for optimizing extremity learning machine by using genetic algorithm
A technology of extreme learning machine and genetic algorithm, applied in the field of computing intelligence and neural network, can solve the problem of poor random parameter over-adaptation, etc., and achieve the effect of improving generalization ability and network stability
- Summary
- Abstract
- Description
- Claims
- Application Information
AI Technical Summary
Problems solved by technology
Method used
Image
Examples
Embodiment Construction
[0024] In order to make the object, technical solution and advantages of the present invention clearer, the present invention will be further described in detail below in conjunction with the accompanying drawings and embodiments. It should be understood that the specific embodiments described here are only used to explain the present invention, not to limit the present invention.
[0025] Such as figure 1 As shown, a kind of integrated frame method using genetic algorithm to optimize extreme learning machine of the present invention comprises the following steps:
[0026] S10: Encode the input weights randomly generated by the extreme learning machine and the hidden layer node thresholds into genetic algorithm individuals, and use the genetic algorithm to randomly generate the initial population.
[0027] Among them, the format of each individual is:
[0028] θ=[w 11 ,w 12 ,...,w 1L ,w 21 ,...w 2L ,w n1 ,...,w nL ,...,b 1 ,b 2 ,...,b L ]
[0029] L is the number ...
PUM
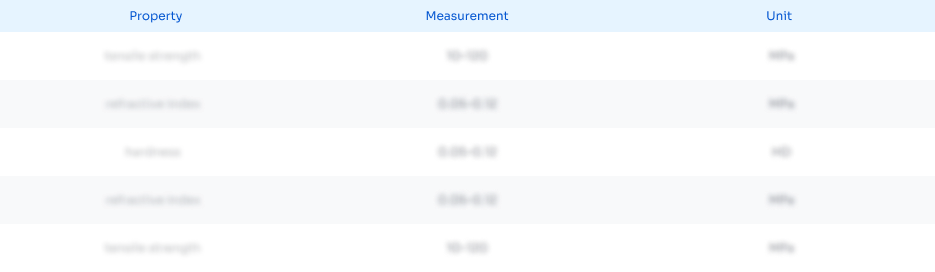
Abstract
Description
Claims
Application Information
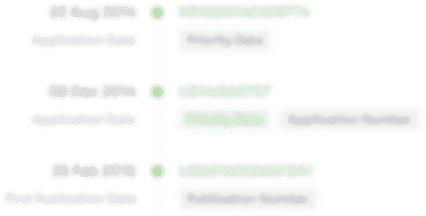
- R&D Engineer
- R&D Manager
- IP Professional
- Industry Leading Data Capabilities
- Powerful AI technology
- Patent DNA Extraction
Browse by: Latest US Patents, China's latest patents, Technical Efficacy Thesaurus, Application Domain, Technology Topic, Popular Technical Reports.
© 2024 PatSnap. All rights reserved.Legal|Privacy policy|Modern Slavery Act Transparency Statement|Sitemap|About US| Contact US: help@patsnap.com