A cross-modal retrieval method that can directly measure the similarity between different modal data
A cross-modal, similarity technology, applied in the direction of electrical digital data processing, special data processing applications, instruments, etc., can solve the retrieval method of few different modal similarities, high dimensionality, and large difference in modal data And other issues
- Summary
- Abstract
- Description
- Claims
- Application Information
AI Technical Summary
Problems solved by technology
Method used
Image
Examples
Embodiment
[0092] Suppose we have 2173 pairs of text and image data with known correspondence, and 693 pairs of text data and image data with unknown correspondence. Examples of pictures and text are as follows figure 2 . First, SIFT features are extracted for all image modality data in the database, and the k-means method is used to cluster to form visual words, and then the features are normalized so that the feature vector representing each image is a unit vector. At the same time, perform part-of-speech tagging on all text modal data in the database, remove non-noun words, retain nouns in the text, use all words that have appeared in the database to form a thesaurus, and count the occurrence of words in the thesaurus separately for each text The number of times, using single-text vocabulary frequency for vectorization, and then normalizing the feature vector, so that the feature vector representing each text is a unit vector.
[0093] Express the paired 2173 pairs of data (features...
PUM
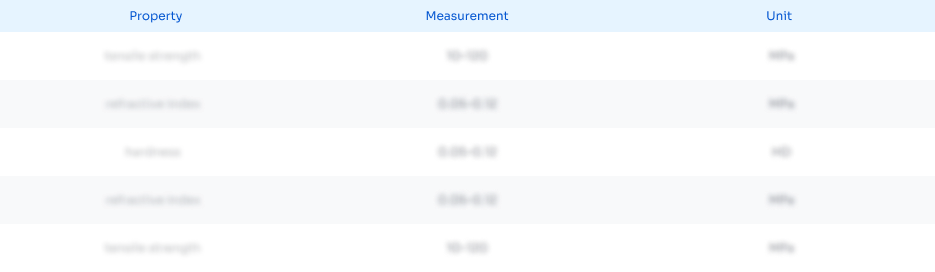
Abstract
Description
Claims
Application Information
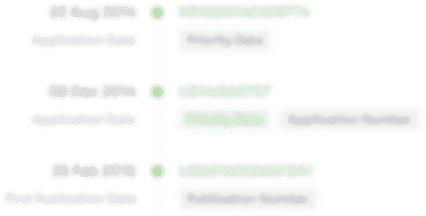
- R&D
- Intellectual Property
- Life Sciences
- Materials
- Tech Scout
- Unparalleled Data Quality
- Higher Quality Content
- 60% Fewer Hallucinations
Browse by: Latest US Patents, China's latest patents, Technical Efficacy Thesaurus, Application Domain, Technology Topic, Popular Technical Reports.
© 2025 PatSnap. All rights reserved.Legal|Privacy policy|Modern Slavery Act Transparency Statement|Sitemap|About US| Contact US: help@patsnap.com