Text categorization method and device
A text classification and text technology, applied in the field of Internet information, can solve the problems of low accuracy, lower accuracy, and affect the accuracy of machine classification, and achieve the effect of improving recognition accuracy and accuracy
- Summary
- Abstract
- Description
- Claims
- Application Information
AI Technical Summary
Problems solved by technology
Method used
Image
Examples
Embodiment 1
[0074] figure 1 Is a flowchart of the text classification method provided in this embodiment, such as figure 1 As shown, the method includes:
[0075] S101. Replace each character in the text to be processed with the exception of characters and numbers with a preset fixed character string.
[0076] First, escape the specific symbols in the text to be processed, such as English symbols "-_`~#$%^&*()+=|\" and Chinese symbols "《》¥()——·?" The characters "\n\t\r\n" and spaces are replaced with fixed characters.
[0077] The fixed character string can be, but is not limited to, using the same characters repeatedly and superimposed into a character string with a length of more than one. For example, a fixed string "$$$$" with four "$" characters superimposed and so on. The fixed character string "$$$$" is used to replace every character in the text to be processed except for words and numbers. For example, for " > > " / " This pending text is replaced with the fixed string "$$$$", and bec...
Embodiment 2
[0095] figure 2 Is a flowchart of the text classification method provided in this embodiment, such as figure 2 As shown, the method includes:
[0096] In step S201, characters other than characters and numbers in the text to be processed are replaced with a preset fixed character string.
[0097] This step is the same as step S101 in the first embodiment, and will not be repeated here.
[0098] Step S202: Count the total length of the replaced text and the length of the text contained in the text, and calculate the text ratio weight by using the ratio of the text length to the total length of the text.
[0099] The calculation method of the ratio K of the text length to the total text length is the same as that of step S102 in the first embodiment, that is, K=L_CHAR / L_ORIG.
[0100] Using the ratio of the text length to the total length of the text to calculate the text ratio weight Score_char, the following formula can be used but not limited to:
Embodiment 3
[0127] In this embodiment, the Bayes dictionary, Fisher dictionary, user name dictionary, and IP dictionary are constructed in advance by the way of offline generation dictionary. The specific establishment methods include:
[0128] Step S301: Obtain sample corpus including normal text and junk text.
[0129] The sample corpus may use a certain scale of existing historical data, and use the text, comments or replies submitted by different user names or IP addresses accumulated in the network to form the sample corpus.
[0130] The normal text and junk text obtained can be classified by using existing classification methods, or it can be obtained by manual labeling, to distinguish the text in the sample corpus that is marked as junk text by the administrator or other users, and Unmarked normal text.
[0131] Step S302: Perform word segmentation processing on the text in the sample corpus, count each word item, calculate the probability that each word item is normal text and junk text, ...
PUM
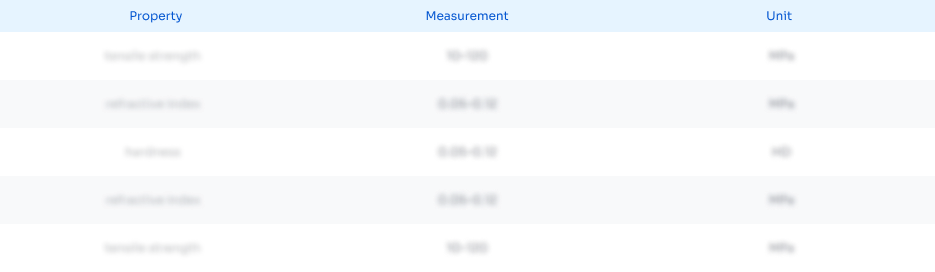
Abstract
Description
Claims
Application Information
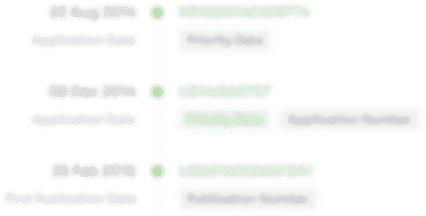
- Generate Ideas
- Intellectual Property
- Life Sciences
- Materials
- Tech Scout
- Unparalleled Data Quality
- Higher Quality Content
- 60% Fewer Hallucinations
Browse by: Latest US Patents, China's latest patents, Technical Efficacy Thesaurus, Application Domain, Technology Topic, Popular Technical Reports.
© 2025 PatSnap. All rights reserved.Legal|Privacy policy|Modern Slavery Act Transparency Statement|Sitemap|About US| Contact US: help@patsnap.com