Deep learning-based clustering method
A technology of deep learning and clustering methods, applied in the direction of neural learning methods, biological neural network models, instruments, etc., can solve problems such as incompetent clustering tasks, large memory consumption, and incompetent big data background environments, etc. The effect of memory consumption
- Summary
- Abstract
- Description
- Claims
- Application Information
AI Technical Summary
Problems solved by technology
Method used
Image
Examples
Embodiment Construction
[0017] In order to make the object, technical solution and advantages of the present invention clearer, the present invention will be described in further detail below in conjunction with specific embodiments and with reference to the accompanying drawings.
[0018] The present invention adopts the nonlinear mapping method of the deep neural network to perform clustering. Traditional clustering mainly includes three parts: spatial mapping, selecting cluster centers and grouping them, and updating cluster centers. On this basis, the present invention first uses the nonlinear mapping of the deep neural network to map the original data to the feature space, then groups in the feature space and calculates the mean value of each group as the cluster center, and on this basis, the objective function of the deep neural network Add intra-class constraints to continue training the network, and finally use the trained network to map the data to the feature space and cluster again until ...
PUM
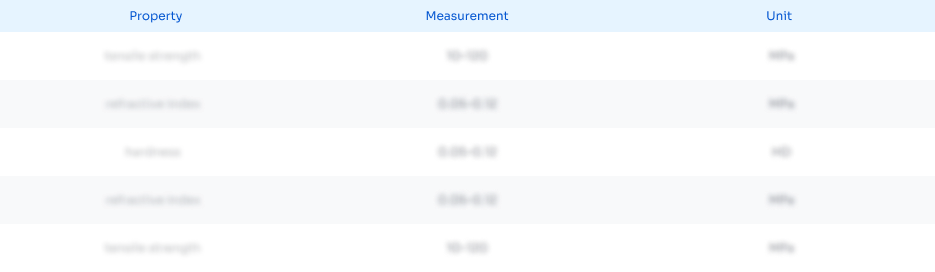
Abstract
Description
Claims
Application Information
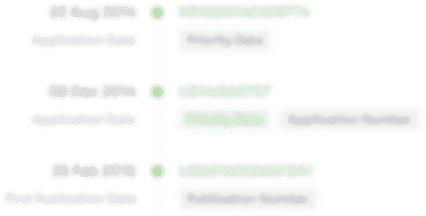
- R&D Engineer
- R&D Manager
- IP Professional
- Industry Leading Data Capabilities
- Powerful AI technology
- Patent DNA Extraction
Browse by: Latest US Patents, China's latest patents, Technical Efficacy Thesaurus, Application Domain, Technology Topic, Popular Technical Reports.
© 2024 PatSnap. All rights reserved.Legal|Privacy policy|Modern Slavery Act Transparency Statement|Sitemap|About US| Contact US: help@patsnap.com