Condition monitoring data stream anomaly detection method based on improved gaussian process regression model
A technology of Gaussian process regression and monitoring data, which is applied in electrical components, wireless communication, etc., and can solve the problem of low abnormality detection effect in processing and monitoring data flow
- Summary
- Abstract
- Description
- Claims
- Application Information
AI Technical Summary
Problems solved by technology
Method used
Image
Examples
specific Embodiment approach 1
[0059] Specific implementation mode one: the abnormal detection method of state monitoring data flow based on the improved Gaussian process regression model of the present embodiment, it comprises the following steps:
[0060] Step 1: According to the obtained offline single-dimensional state monitoring data x, use the autocorrelation analysis method to determine the size of the sliding window of historical data, that is, the value of q, and set the significance level α and the maximum allowable second-type error in the hypothesis test The probability β max ;
[0061] Step 2: Determine the type of the mean function and the covariance function according to the characteristics of the offline single-dimensional state monitoring data x; wherein, the mean function is set as a constant 0, and the covariance function is a combination of a square exponential covariance function and a noise function, where It is defined as follows:
[0062] c ( ...
specific Embodiment approach 2
[0161] Embodiment 2: This embodiment differs from Embodiment 1 in that: in the fifth step, the number of iterations of the iterative search using the conjugate gradient method is 100.
[0162] Other steps and parameters are the same as those in Embodiment 1.
specific Embodiment approach 3
[0163] Specific embodiment three: the difference between this embodiment and specific embodiment one or two is that: in the step six, C(i, i) is the training data D T into the covariance function.
[0164]Other steps and parameters are the same as those in Embodiment 1 or Embodiment 2.
PUM
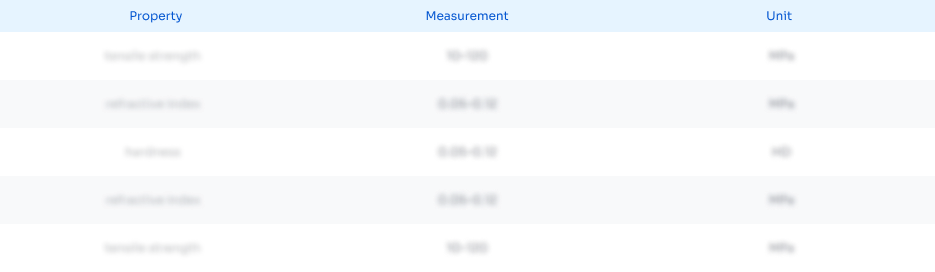
Abstract
Description
Claims
Application Information
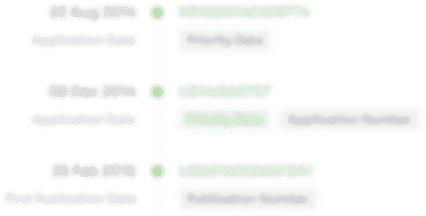
- R&D Engineer
- R&D Manager
- IP Professional
- Industry Leading Data Capabilities
- Powerful AI technology
- Patent DNA Extraction
Browse by: Latest US Patents, China's latest patents, Technical Efficacy Thesaurus, Application Domain, Technology Topic, Popular Technical Reports.
© 2024 PatSnap. All rights reserved.Legal|Privacy policy|Modern Slavery Act Transparency Statement|Sitemap|About US| Contact US: help@patsnap.com