Energy model based image semantic annotation method
A technology of semantic annotation and energy model, which is applied in semantic analysis, image analysis, image data processing, etc., can solve the problems of reduced annotation accuracy, semantic confusion, and failure to consider spatial constraints, so as to avoid semantic confusion and improve accuracy.
- Summary
- Abstract
- Description
- Claims
- Application Information
AI Technical Summary
Problems solved by technology
Method used
Image
Examples
Embodiment Construction
[0078] The present invention will be described in detail below in conjunction with specific embodiments.
[0079] An image semantic annotation method based on an energy model, comprising:
[0080] (1) Divide the original image into several regional images, and extract the visual feature vectors of each regional image.
[0081] In this embodiment, the visual feature fuzzy c-means (FCM) clustering algorithm is used to segment the original image and extract the visual feature vectors of the images in each region. The visual feature vector can be a feature vector based on the Moving Picture Experts Group-7 (MPEG-7) feature, or a feature vector based on the scale-invariant feature transform (Scale-invariant feature transform, SIFT) A feature vector, in this embodiment, is a feature vector based on SIFT features.
[0082] (2) According to the visual feature vector of each region, use the trained SVM classifier to determine the candidate semantic labels of each region image, and th...
PUM
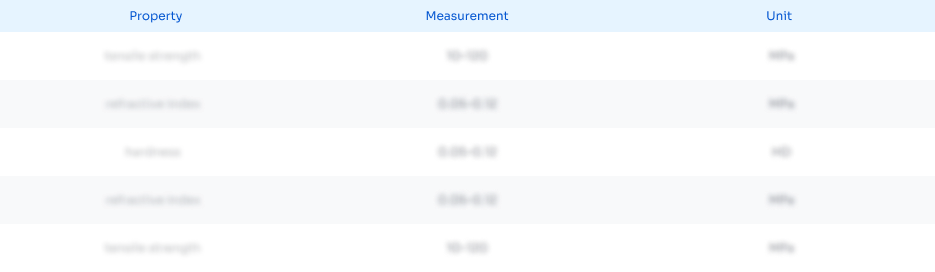
Abstract
Description
Claims
Application Information
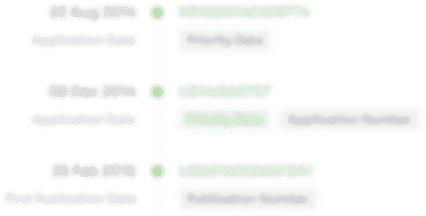
- R&D Engineer
- R&D Manager
- IP Professional
- Industry Leading Data Capabilities
- Powerful AI technology
- Patent DNA Extraction
Browse by: Latest US Patents, China's latest patents, Technical Efficacy Thesaurus, Application Domain, Technology Topic.
© 2024 PatSnap. All rights reserved.Legal|Privacy policy|Modern Slavery Act Transparency Statement|Sitemap