PHD (Probability Hypothesis Density) method for multi-target tracking in uneven clutter environment
A probability hypothesis density, multi-target tracking technology, applied in image data processing, instruments, navigation calculation tools, etc., can solve the problem of inability to achieve fast calculation, large amount of calculation, etc., to achieve improved tracking performance, fast calculation, and simple calculation process Effect
- Summary
- Abstract
- Description
- Claims
- Application Information
AI Technical Summary
Problems solved by technology
Method used
Image
Examples
Embodiment Construction
[0028] The following will clearly and completely describe the technical solutions in the embodiments of the present invention with reference to the accompanying drawings in the embodiments of the present invention. Obviously, the described embodiments are only some, not all, embodiments of the present invention. Based on the embodiments of the present invention, all other embodiments obtained by persons of ordinary skill in the art without making creative efforts belong to the protection scope of the present invention.
[0029] An embodiment of the present invention provides a probability hypothesis density method for multi-target tracking in an uneven clutter environment, such as figure 1 As shown, the method includes the following steps:
[0030] 101. Determine whether the number of echoes accumulated in n frames in the monitoring area is greater than ε.
[0031] Wherein, the n and ε are preset values; the ε is preset by the user according to the number of echoes in the mon...
PUM
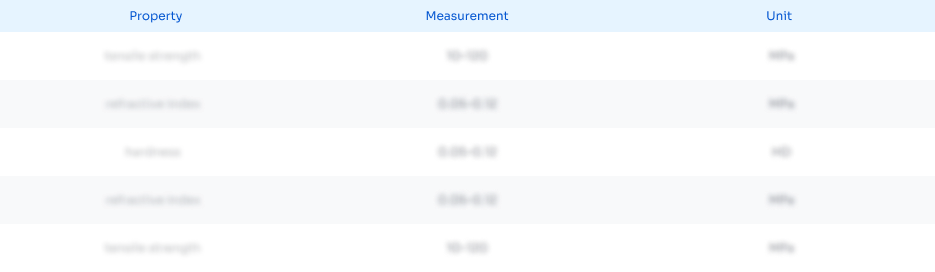
Abstract
Description
Claims
Application Information
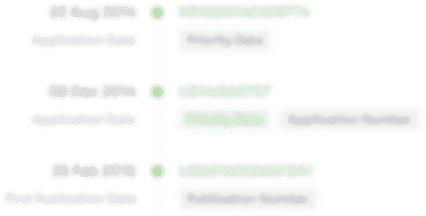
- R&D
- Intellectual Property
- Life Sciences
- Materials
- Tech Scout
- Unparalleled Data Quality
- Higher Quality Content
- 60% Fewer Hallucinations
Browse by: Latest US Patents, China's latest patents, Technical Efficacy Thesaurus, Application Domain, Technology Topic, Popular Technical Reports.
© 2025 PatSnap. All rights reserved.Legal|Privacy policy|Modern Slavery Act Transparency Statement|Sitemap|About US| Contact US: help@patsnap.com