A fpga dynamic power consumption estimation method based on bp neural network
A BP neural network, dynamic power consumption technology, applied in energy-saving computing, software testing/debugging, climate sustainability, etc., can solve problems such as long learning time and learning rate improvement, achieve cost savings, improve work efficiency, guarantee The effect of accuracy
- Summary
- Abstract
- Description
- Claims
- Application Information
AI Technical Summary
Problems solved by technology
Method used
Image
Examples
Embodiment Construction
[0049] The specific embodiments of the present invention will be described in further detail below in conjunction with the accompanying drawings.
[0050] Such as figure 1 As shown, an FPGA dynamic power estimation method based on BP neural network, the dynamic power of FPGA mainly comes from four modules, namely clock tree, programmable resource, I / O (input and output port), block memory, The steps are as follows:
[0051] (1)According to XPE (XPower Estimator), obtain the sample data of the four modules, that is, the input and output of each module; FPGA has many modules, but all include these four modules, and the dynamic power consumption of these four modules is occupied The ratio is relatively large, so the present invention only considers these four modules. The sample data refers to the amount of input and output considered when estimating the power consumption of each module.
[0052] The specific steps for obtaining sample data of the four modules are as follows:
[0053]...
PUM
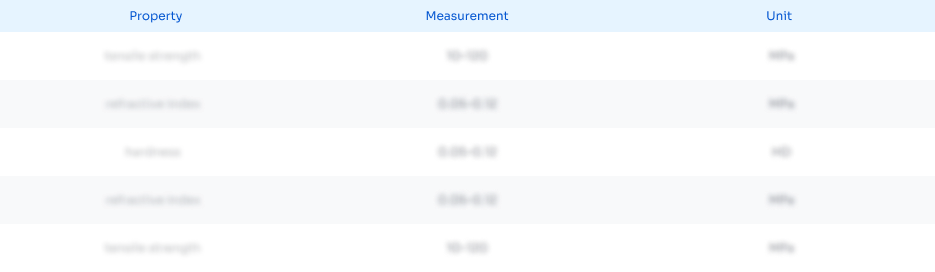
Abstract
Description
Claims
Application Information
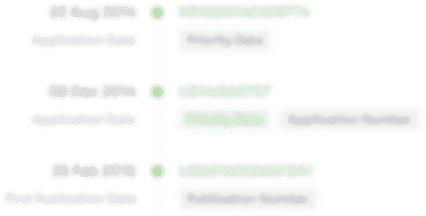
- R&D Engineer
- R&D Manager
- IP Professional
- Industry Leading Data Capabilities
- Powerful AI technology
- Patent DNA Extraction
Browse by: Latest US Patents, China's latest patents, Technical Efficacy Thesaurus, Application Domain, Technology Topic, Popular Technical Reports.
© 2024 PatSnap. All rights reserved.Legal|Privacy policy|Modern Slavery Act Transparency Statement|Sitemap|About US| Contact US: help@patsnap.com