Short-term load predicting method based on quick fuzzy rough set
A short-term load forecasting and fuzzy rough set technology, applied in the field of electric power information, can solve the problems of long time required for attribute reduction and large amount of calculation
- Summary
- Abstract
- Description
- Claims
- Application Information
AI Technical Summary
Problems solved by technology
Method used
Image
Examples
Embodiment 1
[0176] After attribute reduction using the fuzzy rough set method above, 8 conditional attributes with the same classification ability as the original data set can be obtained, namely the maximum temperature on the forecast day, the maximum load 7 days before the forecast date, and the maximum load 1 day before the forecast date. load, the maximum load of the 3 days before the forecast date, the average relative humidity of the 2 days before the forecast date, the date type of the forecast day, the date type of the 2 days before the forecast date and the average relative humidity of the 5 days before the forecast date; Table 2 gives the 8 Attribute importance for reduced attributes.
[0177] Table 2 Condition attribute set obtained after reduction
[0178]
Embodiment 2
[0180] Taking the historical maximum load data of an electric power bureau as the original data and the corresponding data in 2000 and 2001 as the training data, the daily maximum load from March to August 2002 is predicted. The data of the first two weeks of each month is selected as the test set, a total of 6 time periods, and 50 predictions are made in each time period. The BP neural network and the RBF neural network are used for training and prediction, and the evaluation index adopts the absolute value of the average relative error.
[0181] MAPE = 1 N Σ i = 1 N | P A i - P F i | P A ...
PUM
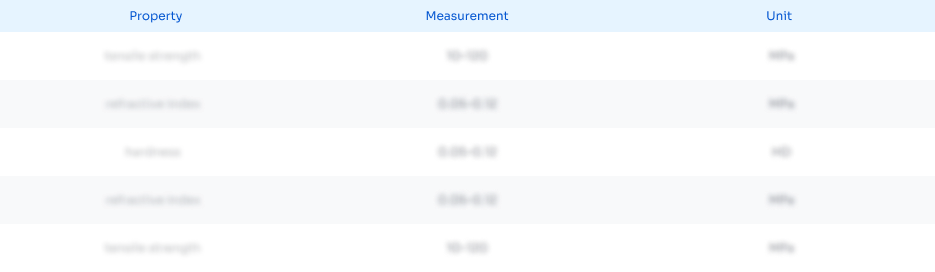
Abstract
Description
Claims
Application Information
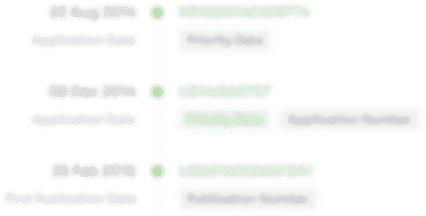
- R&D Engineer
- R&D Manager
- IP Professional
- Industry Leading Data Capabilities
- Powerful AI technology
- Patent DNA Extraction
Browse by: Latest US Patents, China's latest patents, Technical Efficacy Thesaurus, Application Domain, Technology Topic, Popular Technical Reports.
© 2024 PatSnap. All rights reserved.Legal|Privacy policy|Modern Slavery Act Transparency Statement|Sitemap|About US| Contact US: help@patsnap.com