Aluminum plate surface defect classification method based on BP neural network and support vector machine
A BP neural network and support vector machine technology, which is applied in the field of pattern recognition, can solve the problems of increasing hardware complexity, being unfavorable for popularization and use, and increasing costs, and achieves the effect of fast classification, simple and effective implementation, and good classification accuracy.
- Summary
- Abstract
- Description
- Claims
- Application Information
AI Technical Summary
Problems solved by technology
Method used
Image
Examples
Embodiment Construction
[0028] Such as Figure 1-2 As shown, the specific implementation method of this method is illustrated with an example of classification of seven types of cold-rolled aluminum sheet surface defects including oil spots, which mainly includes the following six steps:
[0029] (1) Establish BP neural network model and support vector machine model
[0030] In order to achieve high accuracy classification of oil spots, the remaining defects except oil spots are regarded as the first type of defects, which is the first type of defects.
[0031] The eigenvalues of the oil spots and the eigenvalues of the first type of defects are taken as the input, and the oil spots and the first type of defects are used as the output to construct the BP neural network model, so three layers are selected (namely input layer, hidden layer, output layer ) structure of BP neural network. 24 eigenvalues of defects are extracted from three types of features: gray feature, geometric feature and sha...
PUM
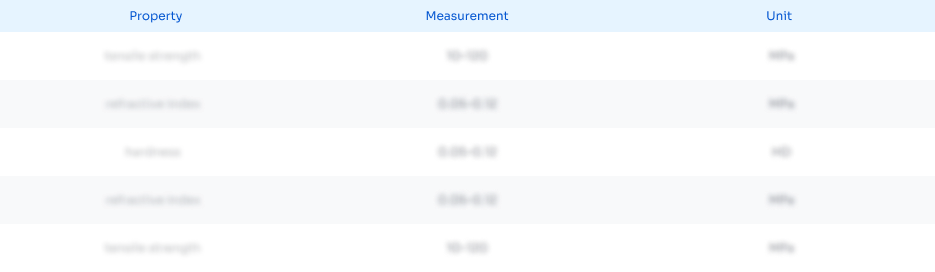
Abstract
Description
Claims
Application Information
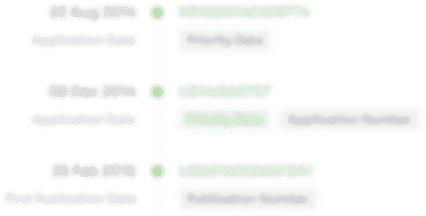
- R&D
- Intellectual Property
- Life Sciences
- Materials
- Tech Scout
- Unparalleled Data Quality
- Higher Quality Content
- 60% Fewer Hallucinations
Browse by: Latest US Patents, China's latest patents, Technical Efficacy Thesaurus, Application Domain, Technology Topic, Popular Technical Reports.
© 2025 PatSnap. All rights reserved.Legal|Privacy policy|Modern Slavery Act Transparency Statement|Sitemap|About US| Contact US: help@patsnap.com