Multi-scale super-pixel hyperspectral remote sensing image classification method for coupling spatial-spectral characteristics
A hyperspectral remote sensing and classification method technology, which is applied in the field of multi-scale super-pixel hyperspectral remote sensing image classification, can solve the problem of low classification accuracy and achieve the effect of improving classification accuracy and improving classification accuracy
- Summary
- Abstract
- Description
- Claims
- Application Information
AI Technical Summary
Problems solved by technology
Method used
Image
Examples
Embodiment approach
[0053] As an implementable manner, the step S101 specifically includes:
[0054] S1011: Set the training set to have a total of n pixels {X 1 ,X 2 …X n}, pixel x i has a label value of Y i (The label value of a certain pixel is used to indicate the feature category of the pixel), and the calculation of any two pixels in the training set X i ,X j The covariance between cov(X i ,X j ), and then get the covariance matrix C of the training set n×n ;
[0055] Specifically, first calculate the mean value of the pixel as Then calculate the different pixels X i ,X j covariance between Finally, the covariance matrix of the training set is obtained
[0056] S1012: Calculate the covariance matrix C n×n The eigenvalue of λ={λ 1 ,λ 2 ,λ 3 ...λ n} and eigenvector E={ξ 1 ,ξ 2 ,ξ 3 …ξ n}; Then select p column vectors corresponding to the first p features with larger eigenvalues in E to construct the pattern matrix E p =[ξ 1 ,ξ 2 ,ξ 3 …ξ p ];
[0057] S1013: P...
PUM
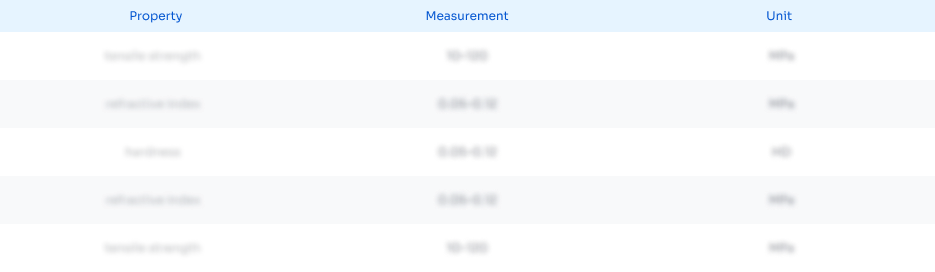
Abstract
Description
Claims
Application Information
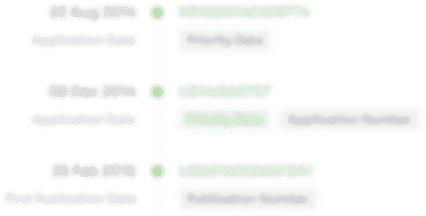
- R&D Engineer
- R&D Manager
- IP Professional
- Industry Leading Data Capabilities
- Powerful AI technology
- Patent DNA Extraction
Browse by: Latest US Patents, China's latest patents, Technical Efficacy Thesaurus, Application Domain, Technology Topic, Popular Technical Reports.
© 2024 PatSnap. All rights reserved.Legal|Privacy policy|Modern Slavery Act Transparency Statement|Sitemap|About US| Contact US: help@patsnap.com