Wind turbine state prediction model establishing method based on grey relation-regression SVM (support vector machine)
A support vector machine and prediction model technology, applied in the field of power grids, can solve problems such as long model training time, many input vectors, and data redundancy
- Summary
- Abstract
- Description
- Claims
- Application Information
AI Technical Summary
Problems solved by technology
Method used
Image
Examples
Embodiment 1
[0088] See figure 1 with figure 2 As shown, a method for establishing a wind turbine state prediction model based on gray relational regression support vector machines of the present invention includes: determining input variables, regression support vector machine training modeling and prediction stages;
[0089] The step of determining the input variable is:
[0090] A1. Collect historical data with forecasting units through the wind power plant SCADA system;
[0091] A2. Select a state quantity that needs to be predicted as the reference sequence, that is, the output; the remaining monitoring items are used as the comparison sequence, that is, the input;
[0092] A3. Calculate the correlation coefficient and degree of correlation between all comparison sequences and reference sequences;
[0093] A4. Arrange the degree of relevance in descending order, and select the monitoring items with greater relevance as the final input for prediction;
[0094] The training modeling steps of the ...
Embodiment 2
[0137] In this example, the SCADA historical record of a single unit of a wind farm in Northeast China that safely operated for one month from March 16 to April 15, 2012 is used as the data source. The system has a total of 44 continuous monitoring items, and the sampling frequency is 1. Times / min.
[0138] (1) Data preprocessing
[0139] The total number of samples in the sampling period is 44600×44 groups, and the data is preprocessed as follows:
[0140] a) When the unit fails, its status and related parameters will fluctuate greatly, which will affect the prediction. Therefore, in order to avoid the impact of wind turbine failure data on the establishment of the model, it is necessary to ensure that there is no unplanned occurrence in the first half month of the selected sample Downtime
[0141] b) The state of wind turbines is inseparable from the wind speed. When the wind speed is too low, the impeller speed is unstable and the unit's operating state is not stable. When the win...
PUM
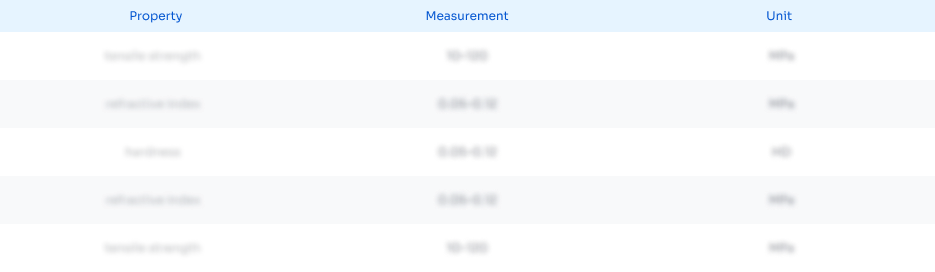
Abstract
Description
Claims
Application Information
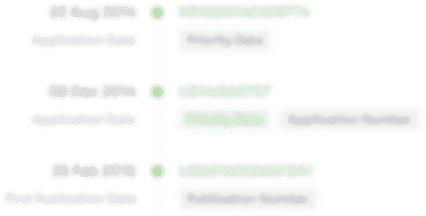
- R&D Engineer
- R&D Manager
- IP Professional
- Industry Leading Data Capabilities
- Powerful AI technology
- Patent DNA Extraction
Browse by: Latest US Patents, China's latest patents, Technical Efficacy Thesaurus, Application Domain, Technology Topic, Popular Technical Reports.
© 2024 PatSnap. All rights reserved.Legal|Privacy policy|Modern Slavery Act Transparency Statement|Sitemap|About US| Contact US: help@patsnap.com