Power interval predication method based on nucleus limit learning machine model
A kernel extreme learning machine and forecasting method technology, applied in forecasting, instrumentation, data processing applications, etc., can solve problems such as unsatisfactory stability and generalization ability, linear inseparability, ELM model output prone to random fluctuations, etc.
- Summary
- Abstract
- Description
- Claims
- Application Information
AI Technical Summary
Problems solved by technology
Method used
Image
Examples
Embodiment Construction
[0039] The embodiments will be described in detail below in conjunction with the accompanying drawings.
[0040] Such as figure 1 As shown in the flow chart, the actual wind power data collected from SCADA by a wind farm in Huaneng Jiuquan during July-October 2014 is used in the present invention, with a resolution of 15 minutes, including the measured output power and the wind speed of the anemometer tower. Interval forecasts for one-day power. The wind farm has 133 1.5MW pitch-adjustable three-blade horizontal-axis asynchronous generators. The method includes the following steps:
[0041] Step 1: Obtain the actual wind speed and power data in the SCADA of the wind farm, process them according to the correlation between wind speed and power, divide the data into training samples and test samples, and normalize the data;
[0042] Step 101: Data preprocessing: collect the one-to-one correspondence data of wind speed and power in SCADA with an interval of 15 minutes, sort the...
PUM
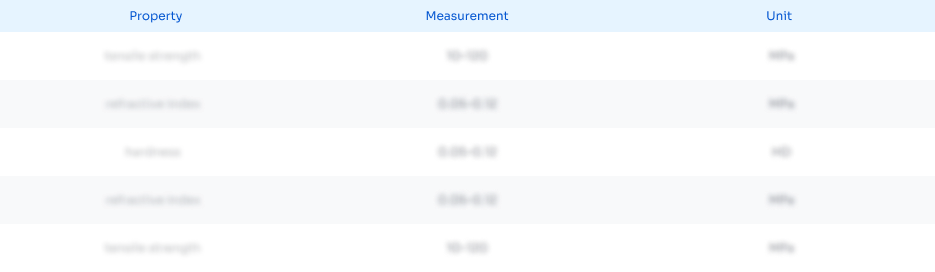
Abstract
Description
Claims
Application Information
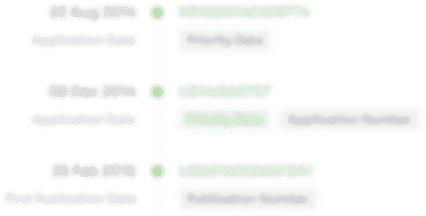
- R&D Engineer
- R&D Manager
- IP Professional
- Industry Leading Data Capabilities
- Powerful AI technology
- Patent DNA Extraction
Browse by: Latest US Patents, China's latest patents, Technical Efficacy Thesaurus, Application Domain, Technology Topic.
© 2024 PatSnap. All rights reserved.Legal|Privacy policy|Modern Slavery Act Transparency Statement|Sitemap