Model combination type speech recognition method based on GMM (Gaussian mixture model) noise estimation
A noise estimation and speech recognition technology, which is applied in speech recognition, speech analysis, instruments, etc., can solve the problems of real-time system acceptance, application range limitation, and noise estimation inability to achieve the effect of saving power and prolonging battery life
- Summary
- Abstract
- Description
- Claims
- Application Information
AI Technical Summary
Problems solved by technology
Method used
Image
Examples
Embodiment Construction
[0015] Below in conjunction with specific embodiment, further illustrate the present invention, should be understood that these embodiments are only used to illustrate the present invention and are not intended to limit the scope of the present invention, after having read the present invention, those skilled in the art will understand various equivalent forms of the present invention All modifications fall within the scope defined by the appended claims of the present application.
[0016] A model combination speech recognition method based on Gaussian mixture model noise estimation, its overall framework is as follows figure 1 shown. The core content of the present invention is the noise estimation module, and its concrete structure is as figure 2 shown. The specific implementations of the noise estimation module and the model combination module are described in detail below.
[0017] 1. Noise estimation
[0018] The present invention only considers the additive backgro...
PUM
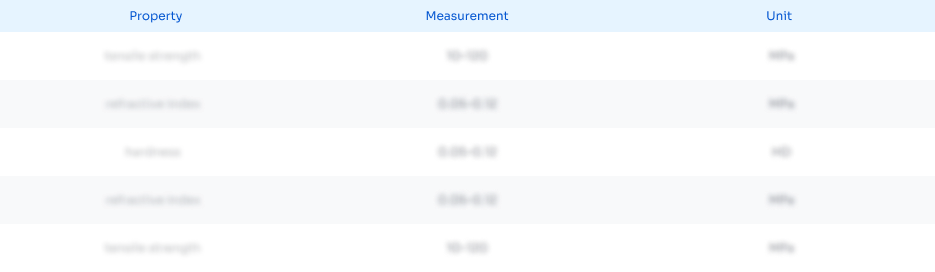
Abstract
Description
Claims
Application Information
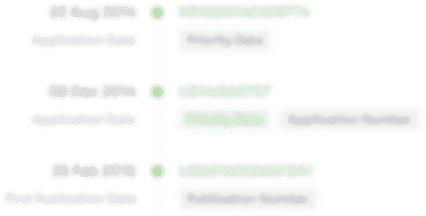
- R&D
- Intellectual Property
- Life Sciences
- Materials
- Tech Scout
- Unparalleled Data Quality
- Higher Quality Content
- 60% Fewer Hallucinations
Browse by: Latest US Patents, China's latest patents, Technical Efficacy Thesaurus, Application Domain, Technology Topic, Popular Technical Reports.
© 2025 PatSnap. All rights reserved.Legal|Privacy policy|Modern Slavery Act Transparency Statement|Sitemap|About US| Contact US: help@patsnap.com