Improved method based on extreme learning machine (ELM) and sparse representation classification
A technology of extreme learning machine and sparse representation, which is applied in the improvement field based on extreme learning machine and sparse representation classification, and can solve the problem of high computational complexity
- Summary
- Abstract
- Description
- Claims
- Application Information
AI Technical Summary
Problems solved by technology
Method used
Image
Examples
Embodiment Construction
[0045] The improvement of the present invention will be verified in conjunction with specific experiments below. The following description is only for demonstration and explanation, and does not limit the present invention in any form.
[0046] Such as figure 1 with figure 2 As shown, to select any database, firstly, a random function randomly generates L hidden layer node parameters (w i ,b i ), i=1,2,…,L, where w i is the input weight connecting the i-th hidden layer node and the input neuron, b i is the deviation of the i-th hidden layer node, and L is the number of hidden layer nodes. Calculate the hidden layer output matrix
[0047] H ( w 1 , ... w L , x 1 , ... , x N , ...
PUM
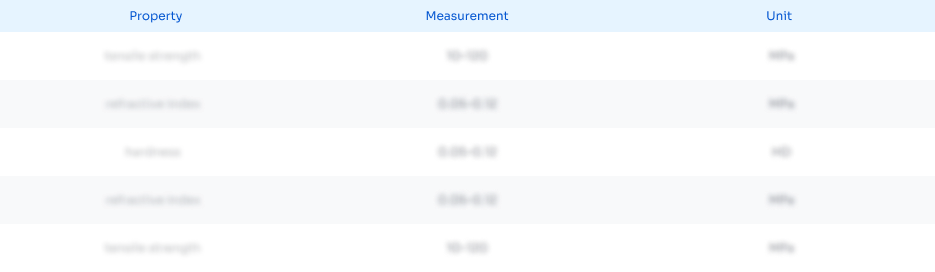
Abstract
Description
Claims
Application Information
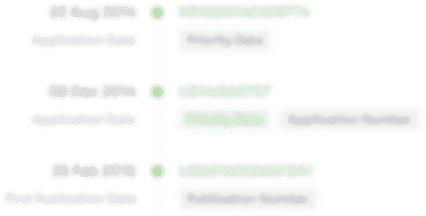
- R&D
- Intellectual Property
- Life Sciences
- Materials
- Tech Scout
- Unparalleled Data Quality
- Higher Quality Content
- 60% Fewer Hallucinations
Browse by: Latest US Patents, China's latest patents, Technical Efficacy Thesaurus, Application Domain, Technology Topic, Popular Technical Reports.
© 2025 PatSnap. All rights reserved.Legal|Privacy policy|Modern Slavery Act Transparency Statement|Sitemap|About US| Contact US: help@patsnap.com