Depth scattering convolution network learning method and system based on nuclear space
A convolutional network and learning method technology, applied in the field of digital image classification schemes, can solve problems such as signal decomposition coefficients not included, image signal classification is inaccurate, etc., to achieve good versatility, strong scalability, and increase the effect of dimensionality
- Summary
- Abstract
- Description
- Claims
- Application Information
AI Technical Summary
Problems solved by technology
Method used
Image
Examples
Embodiment Construction
[0029] The present invention will be described in detail below in conjunction with specific embodiments. The following examples will help those skilled in the art to further understand the present invention, but do not limit the present invention in any form. It should be noted that those skilled in the art can make several modifications and improvements without departing from the concept of the present invention. These all belong to the protection scope of the present invention.
[0030] In order to effectively improve the recognition accuracy of handwritten characters and texture images, the present invention designs a deep scattering convolution network learning method based on kernel space, including:
[0031] Parameterized wavelet generation step: this step constructs a wavelet filter bank with randomized parameters;
[0032] Multi-core learning step: this step decomposes the training data set based on the wavelet filter bank constructed in the parameterized wavelet gen...
PUM
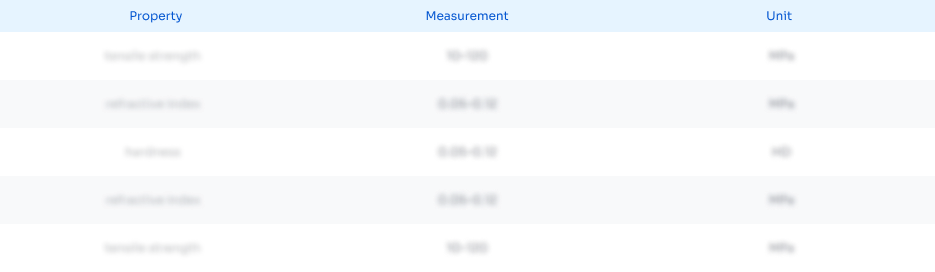
Abstract
Description
Claims
Application Information
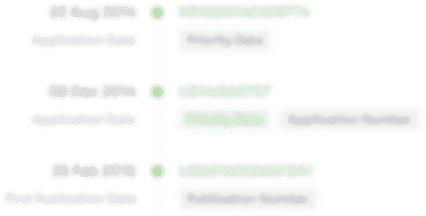
- R&D
- Intellectual Property
- Life Sciences
- Materials
- Tech Scout
- Unparalleled Data Quality
- Higher Quality Content
- 60% Fewer Hallucinations
Browse by: Latest US Patents, China's latest patents, Technical Efficacy Thesaurus, Application Domain, Technology Topic, Popular Technical Reports.
© 2025 PatSnap. All rights reserved.Legal|Privacy policy|Modern Slavery Act Transparency Statement|Sitemap|About US| Contact US: help@patsnap.com