Structure sparsification maintenance based semi-supervised dictionary learning method
A dictionary learning and semi-supervised technology, applied in character and pattern recognition, instruments, computer components, etc., can solve the problems of energy-consuming sample categories, difficulty in obtaining a large number of labeled samples, etc., to achieve proof convergence, fast and effective dictionary The effect of learning, improving sparse representation ability and discriminative ability
- Summary
- Abstract
- Description
- Claims
- Application Information
AI Technical Summary
Problems solved by technology
Method used
Image
Examples
Embodiment Construction
[0032] Below in conjunction with accompanying drawing and embodiment the patent of the present invention is further described.
[0033]The embodiments are only used to further illustrate the present invention, and should not be understood as limiting the protection scope of the present invention. Those skilled in the art can make some non-essential improvements and adjustments to the present invention according to the content of the above invention.
[0034] figure 1 It is the overall flowchart of the present invention. The present invention is a semi-supervised dictionary learning method based on maintaining structure sparsification. The specific operating hardware and programming language of the method of the present invention are not limited, and can be written in any language, so other working modes will not be described in detail.
[0035] Embodiments of the present invention adopt a computer with IntelXeon-E5 central processing unit and 16G byte internal memory, and use...
PUM
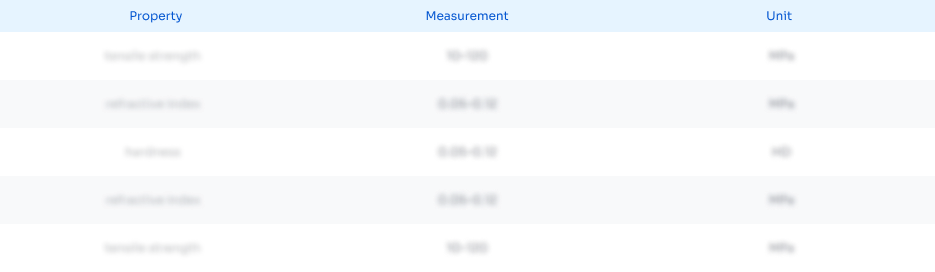
Abstract
Description
Claims
Application Information
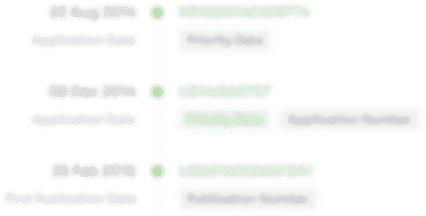
- R&D
- Intellectual Property
- Life Sciences
- Materials
- Tech Scout
- Unparalleled Data Quality
- Higher Quality Content
- 60% Fewer Hallucinations
Browse by: Latest US Patents, China's latest patents, Technical Efficacy Thesaurus, Application Domain, Technology Topic, Popular Technical Reports.
© 2025 PatSnap. All rights reserved.Legal|Privacy policy|Modern Slavery Act Transparency Statement|Sitemap|About US| Contact US: help@patsnap.com