Stock risk prediction method and apparatus
A risk forecasting and stock technology, applied in the field of information processing, can solve problems such as inaccurate stock forecasting and high risk
- Summary
- Abstract
- Description
- Claims
- Application Information
AI Technical Summary
Problems solved by technology
Method used
Image
Examples
Embodiment 1
[0042] refer to figure 1 , showing a stock risk prediction method of the present application, specifically including:
[0043] Step 101: Obtain the stock market field required to be called by the local device.
[0044] The stock market field is an element associated with the stock market. The stock market field that needs to be invoked is set by the local device according to its own broadcast requirements. It can be pre-negotiated and defined by the front-end device and the local device. It is preset information. In the actual application of calling and playing stock market data, the stock market fields to be called can be given once before calling play for the first time, or can be given separately before calling play each time.
[0045] Among them, the stock market fields that need to be called include opening price, closing price, highest price, lowest price, trading volume, turnover, change amount, change rate, return on equity, price-to-book ratio, index smoothing averag...
Embodiment 2
[0062] refer to Figure 4 , showing a stock risk prediction method of the present application, specifically including:
[0063] Step 401: Obtain the stock market field required to be called by the local device.
[0064] Step 402: Predict the stock market field based on the hidden layer of the long-short-term memory recurrent neural network, and obtain the prediction result of the stock, wherein the hidden layer includes an input gate, a forget gate and an output gate.
[0065] Step 402 includes the following sub-steps:
[0066] Step 4021: The input layer of the LSTM recurrent neural network receives the stock market field, and the input layer maps the received stock market field to the hidden layer.
[0067] based on figure 1 The structural block diagram of the long-short-term memory recurrent neural network is shown, and the application shows the internal structure of the hidden layer, specifically as follows Figure 5 As shown, where the hidden layer includes multiple hi...
Embodiment 3
[0091] see Figure 7 , shows a structural block diagram of a stock risk forecasting device in Embodiment 3 of the present application, which may specifically include: an acquisition module 701, configured to acquire the stock market fields required to be called by the local device.
[0092] The prediction module 702 is used to predict the stock market field based on the hidden layer of the long-short-term memory recurrent neural network, and obtain the prediction result of the stock, wherein the prediction result includes the predicted value of the volatility of the stock market on the next trading day, so The long-short-term memory recurrent neural network also includes: an input layer and an output layer.
[0093] The judging module 703 is used for the prediction result is greater than the set threshold, and the stock prediction is risky.
[0094] Preferably, the stock market fields that need to be called include opening price, closing price, highest price, lowest price, tr...
PUM
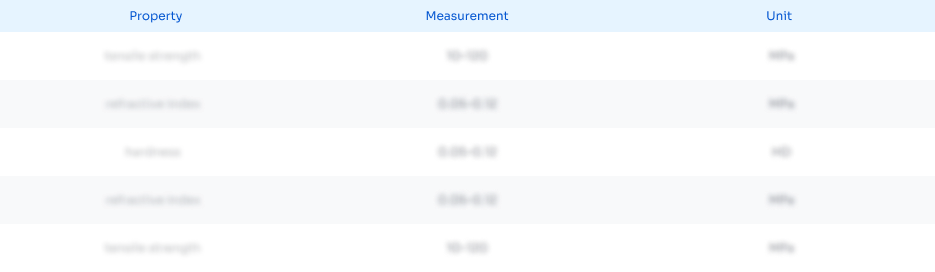
Abstract
Description
Claims
Application Information
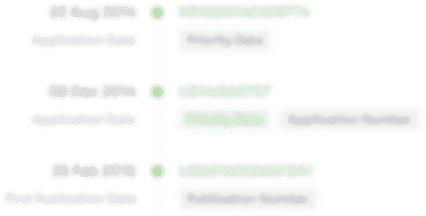
- R&D
- Intellectual Property
- Life Sciences
- Materials
- Tech Scout
- Unparalleled Data Quality
- Higher Quality Content
- 60% Fewer Hallucinations
Browse by: Latest US Patents, China's latest patents, Technical Efficacy Thesaurus, Application Domain, Technology Topic, Popular Technical Reports.
© 2025 PatSnap. All rights reserved.Legal|Privacy policy|Modern Slavery Act Transparency Statement|Sitemap|About US| Contact US: help@patsnap.com