Deep learning-based malicious code sample classification method and system
A malicious code and deep learning technology, applied in the field of information security, can solve the problems of low efficiency and low accuracy, and achieve the effect of overcoming low efficiency, improving accuracy, and improving computing efficiency
- Summary
- Abstract
- Description
- Claims
- Application Information
AI Technical Summary
Problems solved by technology
Method used
Image
Examples
Embodiment Construction
[0044] The present invention provides a malicious code sample classification method and system embodiments based on deep learning, in order to enable those skilled in the art to better understand the technical solutions in the embodiments of the present invention, and to make the above-mentioned purpose and features of the present invention And advantage can be more obvious and easy to understand, below in conjunction with accompanying drawing technical scheme in the present invention is described in further detail:
[0045] The present invention firstly provides an embodiment of a method for classifying malicious code samples based on deep learning, such as figure 1 shown, including:
[0046] S101 disassembles the malicious code sample to obtain assembly code; wherein, the malicious code sample includes but not limited to: apk file or dex file;
[0047]S102 converts the assembly code into image sample data based on the corresponding relationship between the hexadecimal code ...
PUM
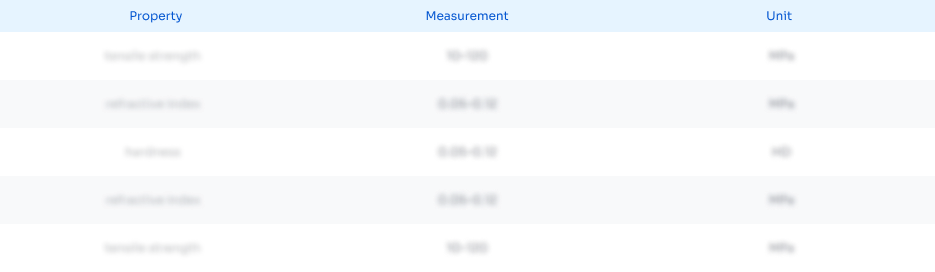
Abstract
Description
Claims
Application Information
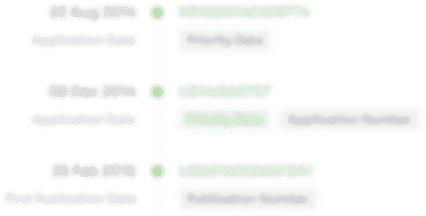
- R&D
- Intellectual Property
- Life Sciences
- Materials
- Tech Scout
- Unparalleled Data Quality
- Higher Quality Content
- 60% Fewer Hallucinations
Browse by: Latest US Patents, China's latest patents, Technical Efficacy Thesaurus, Application Domain, Technology Topic, Popular Technical Reports.
© 2025 PatSnap. All rights reserved.Legal|Privacy policy|Modern Slavery Act Transparency Statement|Sitemap|About US| Contact US: help@patsnap.com