Unsupervised crowd abnormity monitoring and positioning method based on recurrent neural network modeling
A recursive neural network and anomaly monitoring technology, applied in the field of computer vision, can solve the problems of immature crowd abnormal events, inconsistent definitions of basic events, and increased labeling workload, so as to speed up training, overcome gradient disappearance and gradient explosion, The effect of reducing the amount of calculation
- Summary
- Abstract
- Description
- Claims
- Application Information
AI Technical Summary
Problems solved by technology
Method used
Image
Examples
Embodiment Construction
[0030] In order to make the purpose and advantages of the technical solution of the present invention clearer, the present invention will be further described in detail below in conjunction with specific embodiments and with reference to the accompanying drawings.
[0031] Such as figure 1 As shown, the present invention discloses an unsupervised crowd abnormality monitoring and positioning method based on recurrent neural network modeling, and the steps are described in detail as follows.
[0032] (1) Data acquisition: The present invention uses an unsupervised method to learn the temporal and spatial relationship of optical flow features in crowd scenes under normal circumstances, that is, the abnormality is not clearly defined during training, but only captures the characteristics of crowd scenes. The relationship between the optical flow characteristics of crowd dynamic sequences at different times and the optical flow characteristics of local crowd dynamic sequences. If ...
PUM
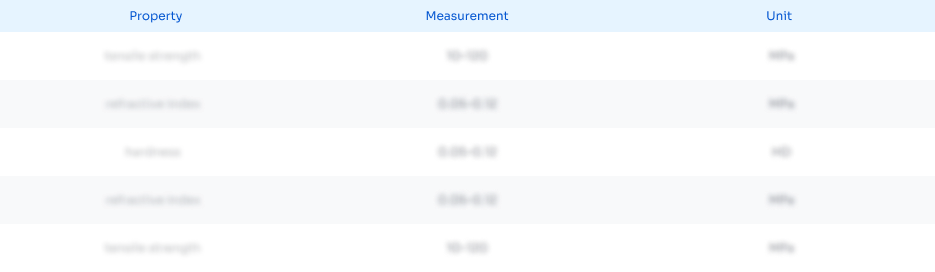
Abstract
Description
Claims
Application Information
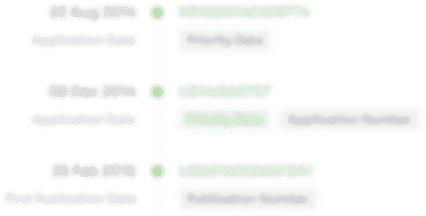
- R&D Engineer
- R&D Manager
- IP Professional
- Industry Leading Data Capabilities
- Powerful AI technology
- Patent DNA Extraction
Browse by: Latest US Patents, China's latest patents, Technical Efficacy Thesaurus, Application Domain, Technology Topic, Popular Technical Reports.
© 2024 PatSnap. All rights reserved.Legal|Privacy policy|Modern Slavery Act Transparency Statement|Sitemap|About US| Contact US: help@patsnap.com