Image attention semantic target segmentation method based on fMRI visual function data DeconvNet
A technology of visual functional data and semantic segmentation, which is applied in the direction of instruments, character and pattern recognition, computer components, etc., can solve problems such as lack of research results, and achieve the effect of improving analysis ability and accuracy
- Summary
- Abstract
- Description
- Claims
- Application Information
AI Technical Summary
Problems solved by technology
Method used
Image
Examples
Embodiment 1
[0017] Embodiment one, see figure 1 As shown, an image attention target semantic segmentation method based on fMRI visual function data DeconvNet includes the following steps:
[0018] Step 1. Collect fMRI visual function data of subjects stimulated by natural scene images, train a deep convolutional neural network model from stimulus images to fMRI visual function data, and a linear mapping from fMRI visual function data to target categories Model, which maps the trained deep convolutional neural network model to a linear mapping model to optimize the network model;
[0019] Step 2. Construct a deconvolution deep network model symmetrical to the deep convolutional neural network optimized by the network model, and optimize the deconvolution deep network model by using the fMRI visual function data and the semantic segmentation results corresponding to the stimulus images. Obtain the mapping from fMRI visual function data to pixel-by-pixel semantic segmentation results, and o...
Embodiment 2
[0023] Embodiment two, see figure 1 As shown, an image attention target semantic segmentation method based on fMRI visual function data DeconvNet includes the following steps:
[0024] Step 1. Collect fMRI visual function data of subjects stimulated by natural scene images, train a deep convolutional neural network model from stimulus images to fMRI visual function data, and a linear mapping from fMRI visual function data to target categories Model, which maps the trained deep convolutional neural network model to a linear mapping model to optimize the network model;
[0025] Step 2. Construct a deconvolution deep network model symmetrical to the deep convolutional neural network optimized by the network model, and optimize the deconvolution deep network model by using the fMRI visual function data and the semantic segmentation results corresponding to the stimulus images. Obtain the mapping from fMRI visual function data to pixel-by-pixel semantic segmentation results, and o...
PUM
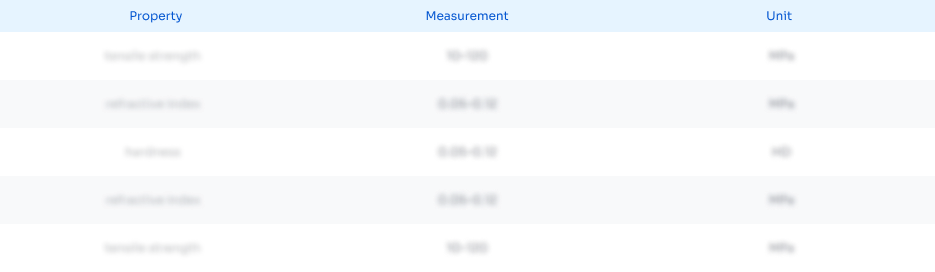
Abstract
Description
Claims
Application Information
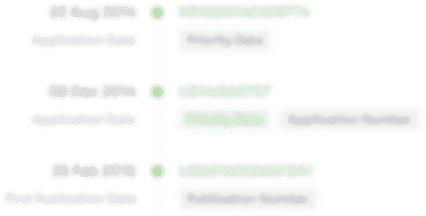
- R&D
- Intellectual Property
- Life Sciences
- Materials
- Tech Scout
- Unparalleled Data Quality
- Higher Quality Content
- 60% Fewer Hallucinations
Browse by: Latest US Patents, China's latest patents, Technical Efficacy Thesaurus, Application Domain, Technology Topic, Popular Technical Reports.
© 2025 PatSnap. All rights reserved.Legal|Privacy policy|Modern Slavery Act Transparency Statement|Sitemap|About US| Contact US: help@patsnap.com