Empirical mode decomposition and Elman neural network combined wind power forecasting method
An empirical mode decomposition and neural network technology, which is applied in the field of wind power forecasting combined with empirical mode decomposition and Elman neural network, can solve problems such as insufficient identification, loss of neural network data, and poor prediction accuracy and prediction speed.
- Summary
- Abstract
- Description
- Claims
- Application Information
AI Technical Summary
Problems solved by technology
Method used
Image
Examples
Embodiment 1
[0111] The present invention is verified by the following Example 1 that the method of the present invention is more accurate and faster than the prior art. Embodiment 1 Based on a specific practical example, the error accuracy of Elman single prediction, empirical mode decomposition and Elman combined prediction is analyzed and compared. The invention has strong fault tolerance and robustness, and has self-learning, self-adaptation, self-organization parallel processing ability and information synthesis ability.
[0112] Forecast the output characteristics of wind farms for three consecutive years, such as figure 2 As shown, according to the analysis of step S1, it is found that the output in spring and winter is the largest, followed by autumn, and the smallest in summer; further using the Pearson correlation analysis formula to calculate the correlation coefficient of annual daily output between the two years, it is found that the positive correlation coefficient of winter...
PUM
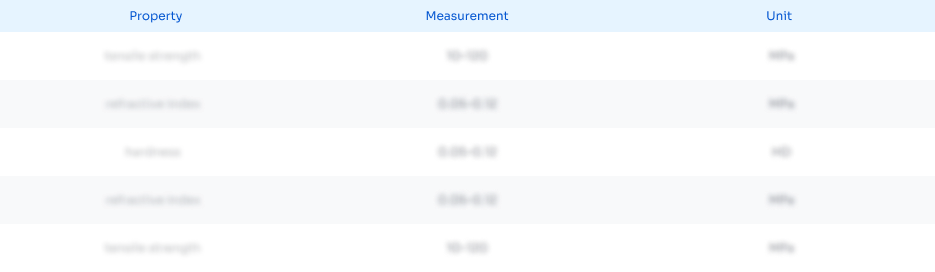
Abstract
Description
Claims
Application Information
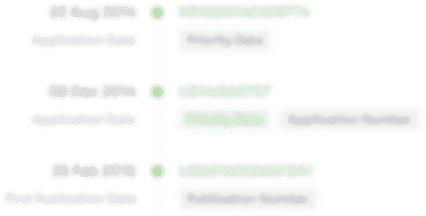
- R&D Engineer
- R&D Manager
- IP Professional
- Industry Leading Data Capabilities
- Powerful AI technology
- Patent DNA Extraction
Browse by: Latest US Patents, China's latest patents, Technical Efficacy Thesaurus, Application Domain, Technology Topic, Popular Technical Reports.
© 2024 PatSnap. All rights reserved.Legal|Privacy policy|Modern Slavery Act Transparency Statement|Sitemap|About US| Contact US: help@patsnap.com