Deep convolution neural network training method and device
A neural network training and deep convolution technology, applied in the field of deep learning, can solve problems such as migration learning that does not involve small-scale target data sets, and achieve the effect of improving migration learning ability, high economic and practical value, and improving prediction ability.
- Summary
- Abstract
- Description
- Claims
- Application Information
AI Technical Summary
Problems solved by technology
Method used
Image
Examples
Embodiment Construction
[0040] In order to make the object, technical solution and advantages of the present invention clearer, the present invention will be further described in detail below in conjunction with the accompanying drawings and embodiments. It should be understood that the specific embodiments described here are only used to explain the present invention, not to limit the present invention.
[0041] The deep convolutional neural network training method and device of the embodiment of the present invention utilizes the advantages of the transfer learning method and the model compression technology to complement each other. During the transfer learning process from a large-scale source data set to a small-scale target data set, the DCNN is model-compressed and Pruning, so as to improve the transfer learning ability, reduce the overfitting risk and deployment difficulty of DCNN on small-scale target data sets, and improve its prediction and recognition rate.
[0042] Specifically, see fig...
PUM
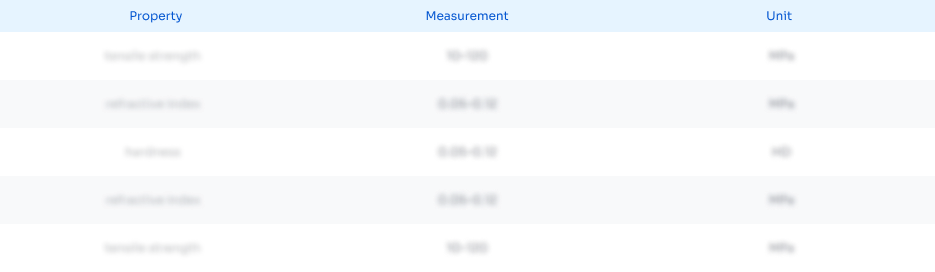
Abstract
Description
Claims
Application Information
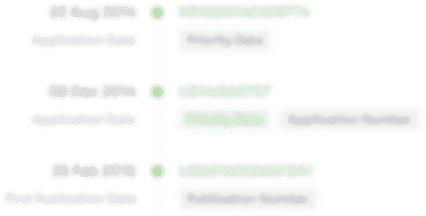
- R&D Engineer
- R&D Manager
- IP Professional
- Industry Leading Data Capabilities
- Powerful AI technology
- Patent DNA Extraction
Browse by: Latest US Patents, China's latest patents, Technical Efficacy Thesaurus, Application Domain, Technology Topic.
© 2024 PatSnap. All rights reserved.Legal|Privacy policy|Modern Slavery Act Transparency Statement|Sitemap