Visual significance detection method based on self-learning characteristics and matrix low-rank recovery
A detection method and feature matrix technology, which are applied in character and pattern recognition, image data processing, instruments, etc., can solve the problems of information redundancy, waste of computing resources, and effectiveness, so as to avoid redundancy, improve sparsity, and save computing. The effect of resources
- Summary
- Abstract
- Description
- Claims
- Application Information
AI Technical Summary
Problems solved by technology
Method used
Image
Examples
Embodiment Construction
[0050] The following will clearly and completely describe the technical solutions in the embodiments of the present invention with reference to the accompanying drawings in the embodiments of the present invention. Obviously, the described embodiments are only some, not all, embodiments of the present invention. Based on the embodiments of the present invention, all other embodiments obtained by persons of ordinary skill in the art without making creative efforts belong to the protection scope of the present invention.
[0051] Such as figure 1 As shown, a visual saliency detection method based on self-learning features and matrix low-rank restoration, the hardware environment used for implementation is: Intel(R) Core(TM) i5CPU 3.2G computer, 8GB memory, 1GB video memory graphics card, running The software environment is: Matlab R2014b and Windows 7. The original image selected in the experiment is a color picture with a resolution of 681*511, such as figure 1 Shown above le...
PUM
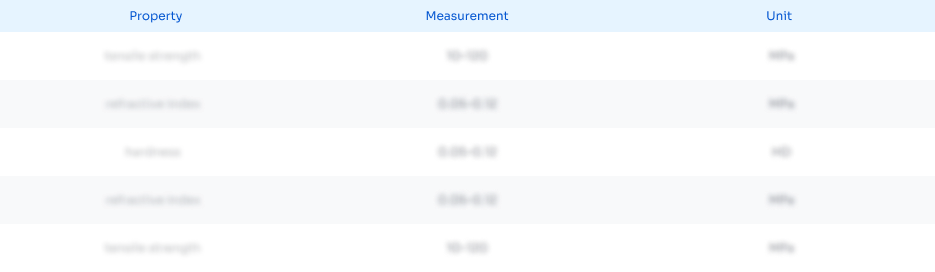
Abstract
Description
Claims
Application Information
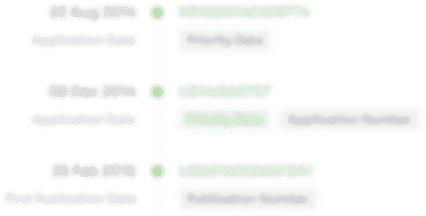
- R&D
- Intellectual Property
- Life Sciences
- Materials
- Tech Scout
- Unparalleled Data Quality
- Higher Quality Content
- 60% Fewer Hallucinations
Browse by: Latest US Patents, China's latest patents, Technical Efficacy Thesaurus, Application Domain, Technology Topic, Popular Technical Reports.
© 2025 PatSnap. All rights reserved.Legal|Privacy policy|Modern Slavery Act Transparency Statement|Sitemap|About US| Contact US: help@patsnap.com