Deep learning-based TFDS non-fault image detection method
A deep learning, non-fault technology, applied in machine learning, instruments, biological neural network models, etc., can solve the problems of low efficiency of automatic fault identification algorithm, easy to miss detection, low practicability, etc., to achieve strong practicability, Avoid missed inspections, mitigate the effects of complex processes
- Summary
- Abstract
- Description
- Claims
- Application Information
AI Technical Summary
Problems solved by technology
Method used
Image
Examples
Embodiment Construction
[0027] The present invention will be further described in detail below in conjunction with the accompanying drawings and specific embodiments.
[0028] figure 1 It is the overall implementation flowchart of the TFDS non-fault detection method based on deep learning of the present invention, such as figure 1 As shown, the fault auxiliary detection method of the present invention comprises the following steps:
[0029] Step 11: Collect TFDS images, establish a training sample library of non-fault images and a test sample library containing fault and non-fault images. Collect the TFDS images of different trains collected on site, and select non-faulty images to build a training sample gallery. The training sample gallery contains all the key areas that need to be detected, and the number of image samples containing different key areas should be basically balanced to avoid sample skew.
[0030] Create a test sample library from faulty and non-faulty images, and prepare multiple ...
PUM
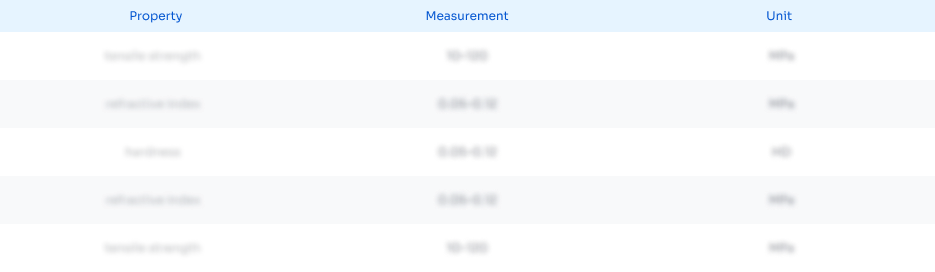
Abstract
Description
Claims
Application Information
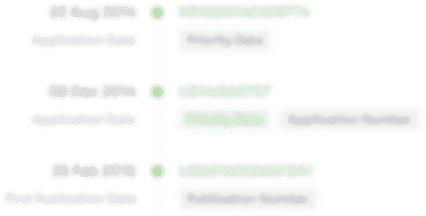
- R&D
- Intellectual Property
- Life Sciences
- Materials
- Tech Scout
- Unparalleled Data Quality
- Higher Quality Content
- 60% Fewer Hallucinations
Browse by: Latest US Patents, China's latest patents, Technical Efficacy Thesaurus, Application Domain, Technology Topic, Popular Technical Reports.
© 2025 PatSnap. All rights reserved.Legal|Privacy policy|Modern Slavery Act Transparency Statement|Sitemap|About US| Contact US: help@patsnap.com