Markov logic network-based knowledge mapping relationship type speculation method and device
A technology of knowledge graph and logical network, applied in reasoning methods, network data retrieval, network data indexing, etc., can solve problems such as poor scalability, incompleteness, and unscientific reasoning rules, and achieve high accuracy and credibility Effect
- Summary
- Abstract
- Description
- Claims
- Application Information
AI Technical Summary
Problems solved by technology
Method used
Image
Examples
Embodiment 1
[0042] Embodiment one, see figure 1 As shown, a Markov logic network-based knowledge graph relationship type inference device includes:
[0043] The inference rule acquisition module is used to generate inference rules according to the path characteristics between the known nodes of the data set knowledge map;
[0044] The credibility weight learning module is used to learn the credibility weight of the inference rules generated by the inference rule acquisition module through the Markov logic network and obtain weighted inference rules;
[0045] The probabilistic inference module is used to perform probabilistic inference on the relationship types between the nodes to be inferred according to the weighted inference rules obtained by the credibility weight learning module, and obtain the probability of the relationship types between the nodes to be inferred;
[0046] The relationship type determination module is used to select a relationship type with a larger probability val...
Embodiment 2
[0048] Embodiment two, see Figure 1~2 As shown, a Markov logic network-based knowledge map relationship type inference method includes the following content:
[0049] Step 1. Based on the knowledge map of the known data set, determine the path characteristics between known nodes and the nodes to be inferred;
[0050] Step 2. Generate inference rules according to the path characteristics between known nodes;
[0051] Step 3. Carry out credibility weight learning on inference rules through Markov logic network to obtain inference rules with weights;
[0052] Step 4. Carry out probabilistic inference on the relationship type between the nodes to be speculated through weighted inference rules;
[0053] Step 5. Determine the relationship type between the nodes to be inferred according to the result of probabilistic reasoning.
[0054] Through the introduction of attitude information, the deviation between the antenna of the Beidou receiver and the GPS receiver is fully considered...
Embodiment 3
[0055] Embodiment three, see Figure 1-7 As shown, a Markov logic network-based knowledge map relationship type inference method includes the following content:
[0056] a. Based on the knowledge graph of the known data set, determine the path characteristics between known nodes and the nodes to be inferred.
[0057] b. Generate inference rules based on the path characteristics between known nodes, including the following content:
[0058] Step b1, use the graph traversal method to traverse the path characteristics between the known nodes of the knowledge graph, generate evidence predicates and query predicates; set the length of the path between known nodes and use the breadth-first traversal method The path features between nodes are traversed to generate evidence predicates and query predicates. The predicates are composed of Figure 4 shown.
[0059] Step b2, according to the evidence predicate and the query predicate, construct an inference rule from the evidence predi...
PUM
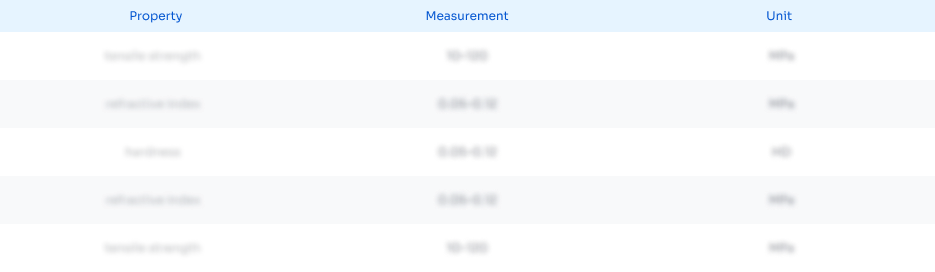
Abstract
Description
Claims
Application Information
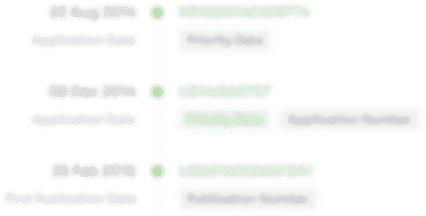
- R&D
- Intellectual Property
- Life Sciences
- Materials
- Tech Scout
- Unparalleled Data Quality
- Higher Quality Content
- 60% Fewer Hallucinations
Browse by: Latest US Patents, China's latest patents, Technical Efficacy Thesaurus, Application Domain, Technology Topic, Popular Technical Reports.
© 2025 PatSnap. All rights reserved.Legal|Privacy policy|Modern Slavery Act Transparency Statement|Sitemap|About US| Contact US: help@patsnap.com