Ganglion differentiation-based incremental target identification system
A target recognition and ganglion technology, applied in the field of target recognition, can solve problems such as poor anti-noise ability and solidified network structure
- Summary
- Abstract
- Description
- Claims
- Application Information
AI Technical Summary
Problems solved by technology
Method used
Image
Examples
Embodiment Construction
[0049] In order to make the present invention clearer and easier to understand, specific embodiments are described in detail below in conjunction with the accompanying drawings.
[0050] Such as figure 1 As shown, the present invention discloses an incremental target recognition system based on ganglion differentiation, which learns online while recognizing, and realizes the growth of the network structure in an unsupervised manner. The method comprises the steps of:
[0051] Step 1, image preprocessing: perform grayscale, normalization and whitening preprocessing on all images in the dataset, such as figure 2 shown.
[0052] Firstly, the color image I is weighted grayscale by the original RGB three-channel according to the ratio of [0.2989, 0.5870, 0.1140] to obtain the image X.
[0053] Next, subtraction normalization is performed, that is: Y ij =X ij -∑ pq w pq ·X i+p,j+q , where w pq is the weight of row p and column q of the Gaussian weighted window, and satisfi...
PUM
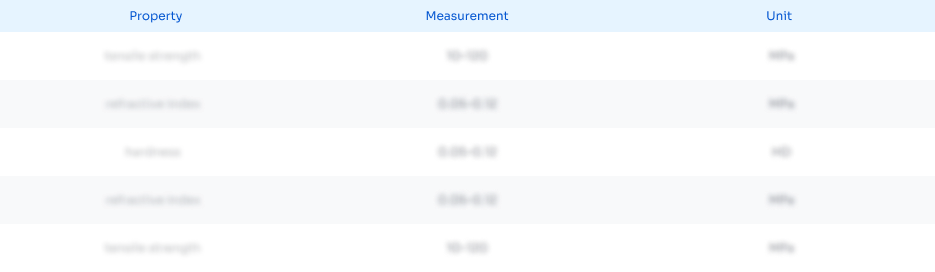
Abstract
Description
Claims
Application Information
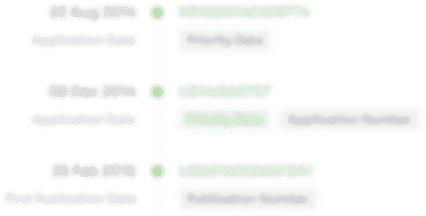
- R&D Engineer
- R&D Manager
- IP Professional
- Industry Leading Data Capabilities
- Powerful AI technology
- Patent DNA Extraction
Browse by: Latest US Patents, China's latest patents, Technical Efficacy Thesaurus, Application Domain, Technology Topic, Popular Technical Reports.
© 2024 PatSnap. All rights reserved.Legal|Privacy policy|Modern Slavery Act Transparency Statement|Sitemap|About US| Contact US: help@patsnap.com