Image super-resolution reconstruction method based on wavelet transformation and convolutional neural network
A convolutional neural network and super-resolution reconstruction technology, which is applied in the field of image super-resolution reconstruction based on wavelet transform and convolutional neural network, can solve the problem that the sparse dictionary model learning ability is not enough to accommodate and cannot realize image super-resolution reconstruction effect and other issues, to achieve the effect of improving the effect of super-resolution reconstruction
- Summary
- Abstract
- Description
- Claims
- Application Information
AI Technical Summary
Problems solved by technology
Method used
Image
Examples
Embodiment Construction
[0013] Exemplary embodiments of the present invention will be described below with reference to the accompanying drawings. In the interest of clarity and conciseness, not all features of an actual implementation are described in this specification. It should be understood, however, that in developing any such practical embodiment, many implementation-specific decisions must be made in order to achieve the developer's specific goals, such as meeting those constraints related to the system and business, and those Restrictions may vary from implementation to implementation. Furthermore, it should also be understood that such a development effort, while potentially complex and time consuming, would be no more than a rational undertaking for those of ordinary skill in the art having the benefit of this disclosure.
[0014] It should also be noted that, in order to avoid obscuring the present invention due to unnecessary details, only the processing steps closely related to the sol...
PUM
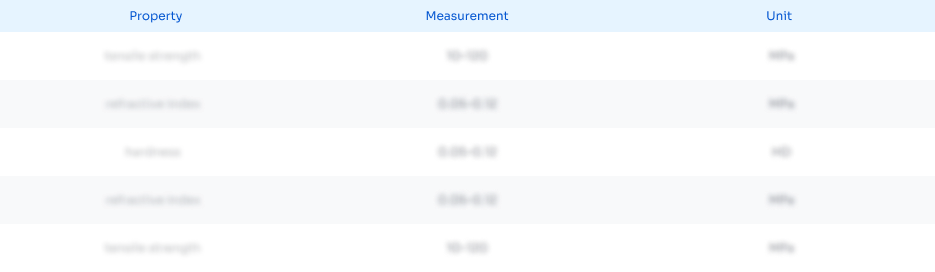
Abstract
Description
Claims
Application Information
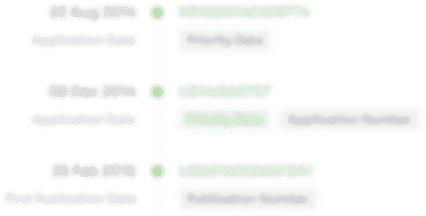
- R&D Engineer
- R&D Manager
- IP Professional
- Industry Leading Data Capabilities
- Powerful AI technology
- Patent DNA Extraction
Browse by: Latest US Patents, China's latest patents, Technical Efficacy Thesaurus, Application Domain, Technology Topic, Popular Technical Reports.
© 2024 PatSnap. All rights reserved.Legal|Privacy policy|Modern Slavery Act Transparency Statement|Sitemap|About US| Contact US: help@patsnap.com