A Generation Method of Routing Metrics Based on Multimodal Data
A multi-modal, data-based technology, applied in relational databases, database models, data processing applications, etc., can solve problems such as inability to provide transmission reliability
- Summary
- Abstract
- Description
- Claims
- Application Information
AI Technical Summary
Problems solved by technology
Method used
Image
Examples
Embodiment Construction
[0026] In order to make the object, technical solution and advantages of the present invention clearer, the present invention will be further described in detail below in conjunction with the accompanying drawings and embodiments. It should be understood that the specific embodiments described here are only used to explain the present invention, not to limit the present invention.
[0027] refer to Figure 1-4 , the invention provides a method for generating routing metrics based on multimodal data, comprising the steps of:
[0028] Step 1: Collect multi-modal data samples, including environment, drivers, vehicles, traffic data, etc. The sources of each modal data are as follows: figure 2 As shown, 201-207 is the source of environmental information, 209-215 is the source of driver information, 217-223 is the source of vehicle information, 225-231 is the source of traffic data information, such as: weather, regional level, buildings, other environments, etc. Several character...
PUM
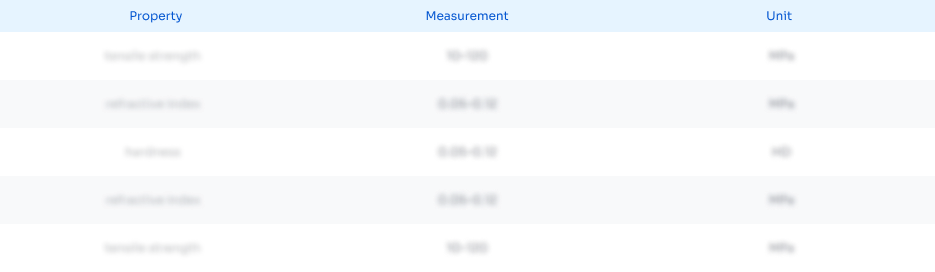
Abstract
Description
Claims
Application Information
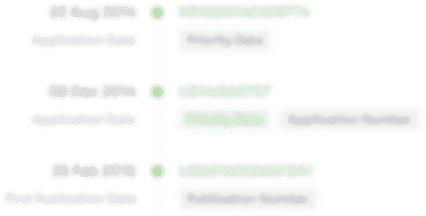
- R&D
- Intellectual Property
- Life Sciences
- Materials
- Tech Scout
- Unparalleled Data Quality
- Higher Quality Content
- 60% Fewer Hallucinations
Browse by: Latest US Patents, China's latest patents, Technical Efficacy Thesaurus, Application Domain, Technology Topic, Popular Technical Reports.
© 2025 PatSnap. All rights reserved.Legal|Privacy policy|Modern Slavery Act Transparency Statement|Sitemap|About US| Contact US: help@patsnap.com