Cross-visual angle gait recognition method based on multitask generation confrontation network
A gait recognition and multi-task technology, applied in the field of machine learning and computer vision, can solve the problem of lack of interpretability of deep models
- Summary
- Abstract
- Description
- Claims
- Application Information
AI Technical Summary
Problems solved by technology
Method used
Image
Examples
experiment example 1
[0081] Experimental example 1: Recognition performance of multi-task generative confrontation network
[0082] This part of the experiment shows different models, recognition accuracy in cross-view. As comparative methods, we chose autoencoders, canonical correlation analysis, linear discriminant analysis, convolutional neural networks, and local tensor discriminant models. Table 1 shows the comparison between the method of the present invention and other methods on three datasets. It can be seen that the present invention has a great improvement compared with other methods.
experiment example 2
[0083] Experimental example 2: Effect of different loss functions on model performance
[0084]Table 2 shows the performance change of the model on the CASIA-B dataset when using different loss functions. It can be seen that combining multi-task confrontation loss and pixel-by-pixel loss can improve the recognition performance of the model; while using different loss functions alone will reduce the performance of the model.
experiment example 3
[0085] Experimental Example 3: Effects of Different Walking States on Model Performance
[0086] image 3 Shows the cross-view recognition accuracy on CASIA-B in different walking states. There are three different walking states: normal walking, walking with a bag, and walking with a coat. As can be seen from the figure, the accuracy rate is the highest when walking normally, and the gait sequence wearing a jacket has a more significant reduction in model performance than the gait sequence carrying a bag.
PUM
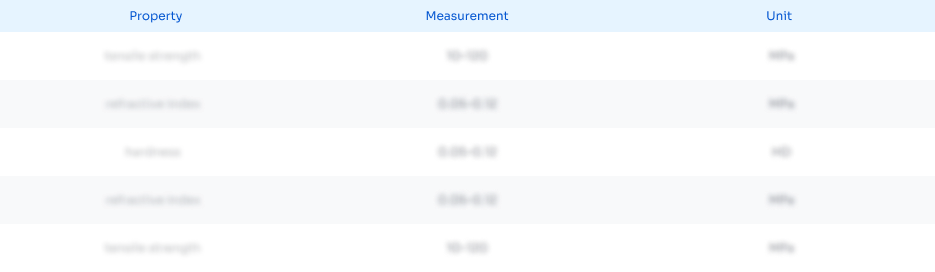
Abstract
Description
Claims
Application Information
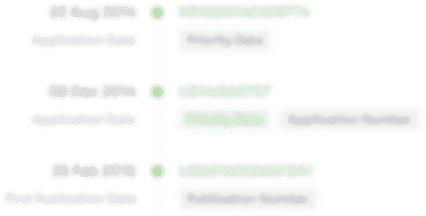
- R&D Engineer
- R&D Manager
- IP Professional
- Industry Leading Data Capabilities
- Powerful AI technology
- Patent DNA Extraction
Browse by: Latest US Patents, China's latest patents, Technical Efficacy Thesaurus, Application Domain, Technology Topic, Popular Technical Reports.
© 2024 PatSnap. All rights reserved.Legal|Privacy policy|Modern Slavery Act Transparency Statement|Sitemap|About US| Contact US: help@patsnap.com