Depth feature extraction method for three-dimensional model
A 3D model and deep feature technology, applied in 3D object recognition, character and pattern recognition, instruments, etc., can solve problems such as large memory storage space, long network learning time, and inability to fully express 3D model information
- Summary
- Abstract
- Description
- Claims
- Application Information
AI Technical Summary
Problems solved by technology
Method used
Image
Examples
Embodiment
[0064] Such as Figure 1 to Figure 4 As shown, the depth feature extraction method of the three-dimensional model of the present invention is as follows:
[0065] First, extract the polar view of the 3D model as the training input data for the deep convolutional neural network;
[0066] Secondly, construct a deep convolutional neural network and train the polar view; among them, the deep convolutional neural network includes an input layer with the polar view as the training input data, which is used to learn the features of the polar view and obtain a two-dimensional feature map The convolutional layer, the pooling layer used to aggregate the two-dimensional feature maps of different positions and reduce the feature dimension, the fully connected layer used to arrange and link the two-dimensional feature maps to form a one-dimensional vector, and the output The output layer of the category prediction result;
[0067] Again, input the polar view into the deep convolutional n...
PUM
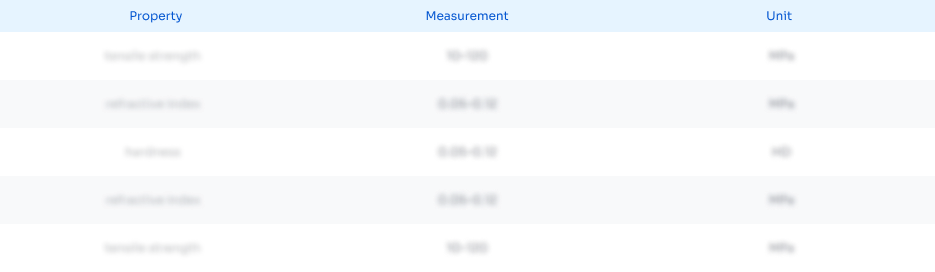
Abstract
Description
Claims
Application Information
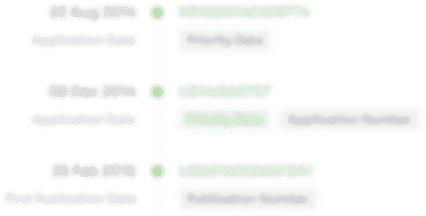
- R&D Engineer
- R&D Manager
- IP Professional
- Industry Leading Data Capabilities
- Powerful AI technology
- Patent DNA Extraction
Browse by: Latest US Patents, China's latest patents, Technical Efficacy Thesaurus, Application Domain, Technology Topic, Popular Technical Reports.
© 2024 PatSnap. All rights reserved.Legal|Privacy policy|Modern Slavery Act Transparency Statement|Sitemap|About US| Contact US: help@patsnap.com