Road network state prediction method based on depth space-time convolution circulation network
A prediction method and road network technology, applied in biological neural network models, traffic flow detection, neural architecture, etc., can solve problems such as difficulty in considering the spatial relationship of different road sections, trunk roads, and limited prediction scope.
- Summary
- Abstract
- Description
- Claims
- Application Information
AI Technical Summary
Problems solved by technology
Method used
Image
Examples
Embodiment 1
[0053] In this embodiment, the data used is a certain road network in Beijing provided by a certain company, and the data includes 9 fields. As shown in Table 1, the road section data is updated every 2 minutes, wherein the data fields directly related to the present invention include There are three fields of time, section number, and speed, the time span is 3 months, and the number of sections is 278.
[0054]
[0055] The realization route of the present invention comprises the following steps:
[0056] Step 1: Establish a sample set, divide the training set and test set, and select a road network such as figure 1 As shown in A, the road network is divided into various small road sections, including 278 road sections in total, and each road section is numbered, recorded as (1,2,3,...,278), and 24 hours a day is divided into each time length. Time period (every 2 minutes as a time period).
[0057] First, calculate the average speed of each road segment at each time per...
PUM
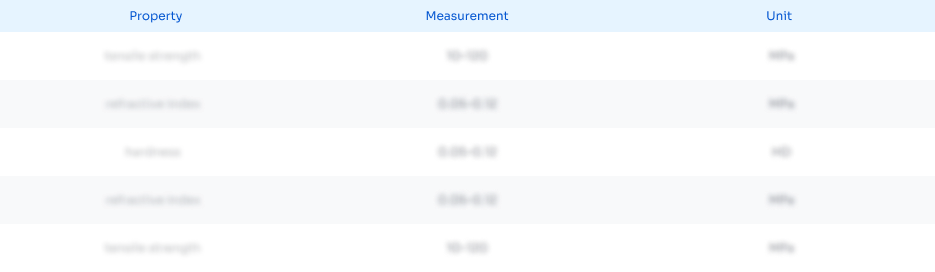
Abstract
Description
Claims
Application Information
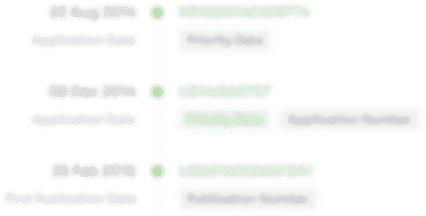
- R&D
- Intellectual Property
- Life Sciences
- Materials
- Tech Scout
- Unparalleled Data Quality
- Higher Quality Content
- 60% Fewer Hallucinations
Browse by: Latest US Patents, China's latest patents, Technical Efficacy Thesaurus, Application Domain, Technology Topic, Popular Technical Reports.
© 2025 PatSnap. All rights reserved.Legal|Privacy policy|Modern Slavery Act Transparency Statement|Sitemap|About US| Contact US: help@patsnap.com