Short-term wind speed multi-step prediction method based on deep learning method
A deep learning and multi-step forecasting technology, applied in neural learning methods, biological neural network models, etc., can solve problems such as poor wind speed forecasting effect
- Summary
- Abstract
- Description
- Claims
- Application Information
AI Technical Summary
Problems solved by technology
Method used
Image
Examples
specific Embodiment approach 1
[0021] Specific implementation mode one: combine figure 1 Describe this embodiment, the short-term wind speed multi-step prediction method based on the deep learning method described in this embodiment,
[0022] Step 1: Based on the deep learning method, establish a deep neural network regression model with a multi-input multi-output structure;
[0023] Step 2: Using the layer-by-layer greedy method, combined with the recent actual wind speed data of the measured wind farm, the deep neural network regression model established in step 1 is trained, and through the nonlinear mapping function of the model, the sequence of the model is obtained through learning. The mapping relationship between them is used to determine the deep neural network regression model;
[0024] Step 3: According to the deep neural network regression model determined in step 2, perform multi-step prediction on the actual wind speed of the measured wind farm, and obtain the wind speed prediction result of ...
specific Embodiment approach 2
[0025] Embodiment 2: This embodiment is a further limitation of the short-term wind speed multi-step prediction method based on the deep learning method described in Embodiment 1.
[0026] In the described step one, based on the deep learning method, the method for setting up a deep neural network regression model with a multi-input multi-output structure is:
[0027] Constructing a deep neural network regression model with a multiple-input multiple-output structure by stacking restricted Boltzmann machines (RBMs),
[0028] The deep neural network regression model with the multi-input multi-output structure is an l-layer neural network, and the vector x=h 0 represents the original input, (h 1 ,...,h l-1 ) represents the input of the corresponding hidden layer, h l Represents the input of the output layer; its 1:l-1 hidden layer uses a sigmoid function and is composed of a restricted Boltzmann machine, and the top activation function uses a pure linear function;
[0029] Fo...
specific Embodiment approach 3
[0075] Embodiment 3: This embodiment is a further limitation of the short-term wind speed multi-step prediction method based on the deep learning method described in Embodiment 1.
[0076] Using the layer-by-layer greedy method, combined with the actual wind speed data of the measured wind farm, the deep neural network regression model established in step 1 is trained, and through the nonlinear mapping function of the model, the mapping relationship between the sequences of the model is obtained through learning. , to determine the process of deep neural network regression model as;
[0077] Step 21: Layer the deep neural network regression model established in step 1, from bottom to top, and then use the actual wind speed data of the measured wind farm to perform unsupervised training on the layer that inputs x;
[0078] Step 22: After the unsupervised training is over, supervised learning is used to fine-tune the deep neural network regression model:
[0079] In addition to...
PUM
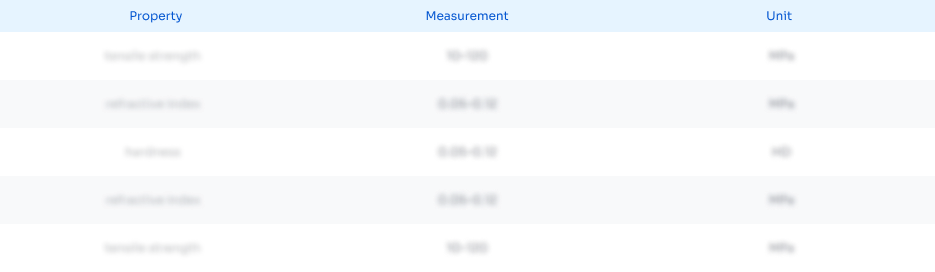
Abstract
Description
Claims
Application Information
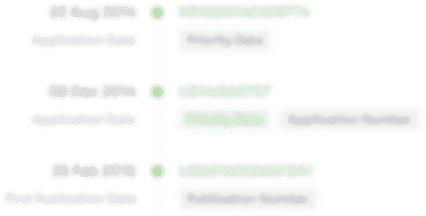
- R&D
- Intellectual Property
- Life Sciences
- Materials
- Tech Scout
- Unparalleled Data Quality
- Higher Quality Content
- 60% Fewer Hallucinations
Browse by: Latest US Patents, China's latest patents, Technical Efficacy Thesaurus, Application Domain, Technology Topic, Popular Technical Reports.
© 2025 PatSnap. All rights reserved.Legal|Privacy policy|Modern Slavery Act Transparency Statement|Sitemap|About US| Contact US: help@patsnap.com