Preceding vehicle following method based on deep convolutional neutral network
A neural network and deep convolution technology, applied in the field of following vehicles based on deep convolutional neural networks, can solve the cumbersome visual ranging and controller design process of the adaptive cruise system, cannot meet different personal driving habits, and cannot be fine-tuned Control parameters and other issues to achieve the effect of avoiding visual distance measurement and controller design process, reducing theoretical basis requirements, and universal application range
- Summary
- Abstract
- Description
- Claims
- Application Information
AI Technical Summary
Problems solved by technology
Method used
Image
Examples
Embodiment
[0044] In this embodiment, a car following method based on a deep convolutional neural network, such as figure 1 shown, including the following steps:
[0045] S1. Establish a training sample library; the specific process includes:
[0046] S11. Use the experimental vehicle to collect driving training data in different locations, climates, and weathers. The training data includes the visual data of the forward-looking camera and the corresponding driving manipulation data. The manipulation data includes the sensor data of the accelerator pedal and the automatic pedal;
[0047] S12. Synchronizing the visual data and the manipulation data;
[0048] S13. Screen the data of the car-following operation part, discretize the data, generate data samples, and complete the establishment of the training sample library.
[0049]S2. Establish a deep convolutional neural network; a deep convolutional neural network is generally composed of convolution, pooling, and full connection operati...
PUM
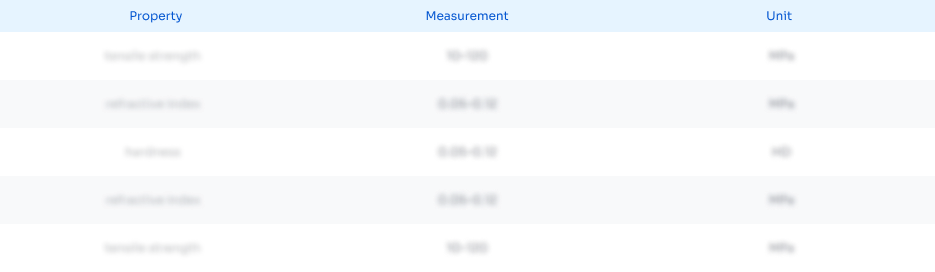
Abstract
Description
Claims
Application Information
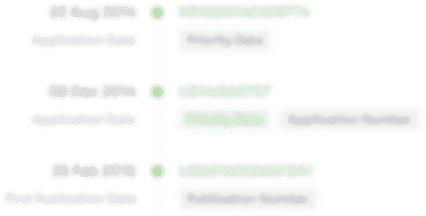
- R&D Engineer
- R&D Manager
- IP Professional
- Industry Leading Data Capabilities
- Powerful AI technology
- Patent DNA Extraction
Browse by: Latest US Patents, China's latest patents, Technical Efficacy Thesaurus, Application Domain, Technology Topic.
© 2024 PatSnap. All rights reserved.Legal|Privacy policy|Modern Slavery Act Transparency Statement|Sitemap