Expressway unmanned vehicle formation method based on multi-agent reinforcement learning
An unmanned vehicle and reinforcement learning technology, applied in the field of intelligent vehicles, can solve the problems of poor stability of the formation system, limited vehicle perception, and high stability requirements, and achieve enhanced stability and fault tolerance, increased control constraints, and training difficulty big effect
- Summary
- Abstract
- Description
- Claims
- Application Information
AI Technical Summary
Problems solved by technology
Method used
Image
Examples
Embodiment 1
[0050] Such as figure 1 As shown, the present invention relates to a high-speed road unmanned vehicle formation method based on multi-agent reinforcement learning, which obtains environmental information as observation input into the trained Q-MIX network, obtains the action decision of each unmanned vehicle, and realizes the formation , wherein, the training method of Q-MIX network comprises the following steps:
[0051] S1: Initialize the training environment.
[0052] S2: Input the environmental information of the training environment into the Q-MIX network as observations to obtain the action decisions of each unmanned vehicle, that is, to obtain the decision-making strategy adopted by each unmanned vehicle facing the current scene, which is divided into: change lanes to the left , lane keeping, change lane to the right.
[0053] Further, the observations made of environmental information include local observations and global observations, wherein the local observations ...
Embodiment 2
[0084] This implementation case provides a method for formation decision-making of unmanned vehicles on high-speed roads based on multi-agent reinforcement learning. The method framework is as follows: Figure 4 shown. This method divides the decision-making control into two parts. The first part inputs the environmental information as an observation into the QMIX network, and outputs the current decision of each formation vehicle (lane change to the left, lane keeping, and lane change to the right). The second part is based on Decision-making information, trajectory planning, and calculation of control variables (acceleration, direction). The reward obtained by the vehicle for performing this action is the reward value of QMIX. After training, an intelligent vehicle formation decision model in high-speed scenarios can be obtained. That is to say, the present invention trains a set of decision-making and control strategies for intelligent vehicle formations on expressways th...
PUM
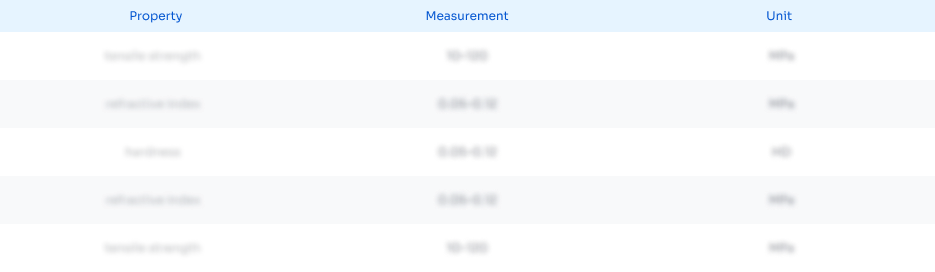
Abstract
Description
Claims
Application Information
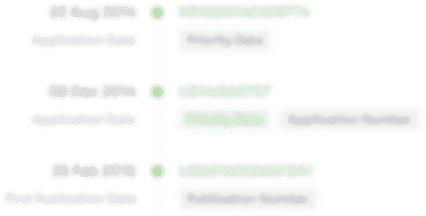
- R&D Engineer
- R&D Manager
- IP Professional
- Industry Leading Data Capabilities
- Powerful AI technology
- Patent DNA Extraction
Browse by: Latest US Patents, China's latest patents, Technical Efficacy Thesaurus, Application Domain, Technology Topic, Popular Technical Reports.
© 2024 PatSnap. All rights reserved.Legal|Privacy policy|Modern Slavery Act Transparency Statement|Sitemap|About US| Contact US: help@patsnap.com