Image classification method and device
A classification method and image technology, applied in the field of transfer learning and deep learning, can solve the problem of insufficient image feature extraction and image classification rate
- Summary
- Abstract
- Description
- Claims
- Application Information
AI Technical Summary
Problems solved by technology
Method used
Image
Examples
specific Embodiment approach
[0103] As a specific implementation, the above-mentioned hybrid model training module may include:
[0104] An acquisition unit, configured to acquire a small image data set, and input the small data set into the migration model structure;
[0105] The first extraction unit is used to extract the initial features of the small image data set by using the underlying feature extraction layer;
[0106] A feature training unit, configured to use the residual network layer to train the initial features to obtain the first feature map;
[0107] The second extraction unit is used to extract the second feature map of the image small data set by using the multi-scale pooling layer;
[0108] A concatenation unit, configured to concatenate the first feature map and the second feature map to obtain the target feature map;
[0109] The parameter update training unit is used to input the target feature map into the softmax classifier, and use the batch gradient descent method to iterativel...
PUM
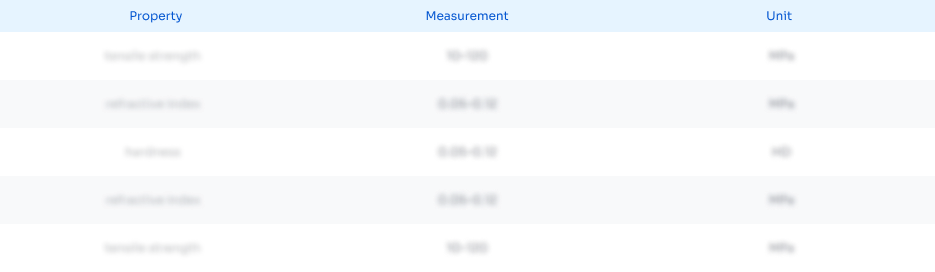
Abstract
Description
Claims
Application Information
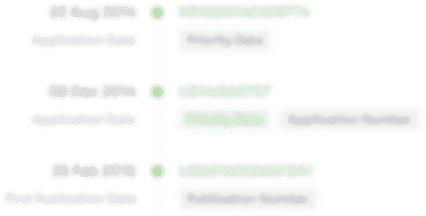
- R&D
- Intellectual Property
- Life Sciences
- Materials
- Tech Scout
- Unparalleled Data Quality
- Higher Quality Content
- 60% Fewer Hallucinations
Browse by: Latest US Patents, China's latest patents, Technical Efficacy Thesaurus, Application Domain, Technology Topic, Popular Technical Reports.
© 2025 PatSnap. All rights reserved.Legal|Privacy policy|Modern Slavery Act Transparency Statement|Sitemap|About US| Contact US: help@patsnap.com