Pulmonary nodule diagnosis method based on local receptive field and semi-supervised depth self coding
A diagnostic method and receptive field technology, applied to radiological diagnostic instruments, diagnostics, computer components, etc., can solve problems such as missed diagnosis and misdiagnosis, and achieve the effect of improving accuracy, increasing accuracy rate, and increasing precision
- Summary
- Abstract
- Description
- Claims
- Application Information
AI Technical Summary
Problems solved by technology
Method used
Image
Examples
Embodiment Construction
[0059] The technical solution of the present invention will be further described in detail below in conjunction with the drawings and specific embodiments.
[0060] The overall detection process diagram of lung nodules is as follows figure 1 As shown, the present invention uses local receptive fields to extract hierarchically different types of features hidden in lung parenchymal CT images, and then uses an improved stacked sparse auto-encoding network that incorporates medically relevant lung disease information. The label's semi-supervised feature extraction depth model is trained to find higher-level features from the CT feature images that have been feature-classified, replace the top-level output layer with a logistic regression classifier, and use the training optimized features as the output vector. Finally, a variety of clinical information is integrated to achieve accurate detection of lung nodules.
[0061] A. Multi-feature learning based on local receptive fields
[0062...
PUM
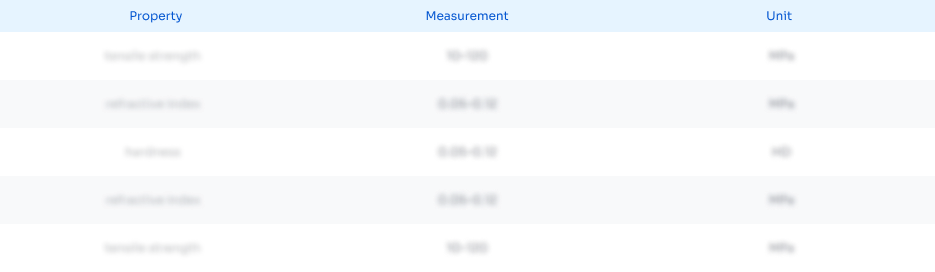
Abstract
Description
Claims
Application Information
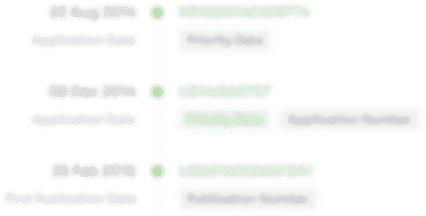
- R&D Engineer
- R&D Manager
- IP Professional
- Industry Leading Data Capabilities
- Powerful AI technology
- Patent DNA Extraction
Browse by: Latest US Patents, China's latest patents, Technical Efficacy Thesaurus, Application Domain, Technology Topic, Popular Technical Reports.
© 2024 PatSnap. All rights reserved.Legal|Privacy policy|Modern Slavery Act Transparency Statement|Sitemap|About US| Contact US: help@patsnap.com